Gunnar König
Paper download is intended for registered attendees only, and is
subjected to the IEEE Copyright Policy. Any other use is strongly forbidden.
Papers from this author
Relative Feature Importance
Gunnar König, Christoph Molnar, Bernd Bischl, Moritz Grosse-Wentrup
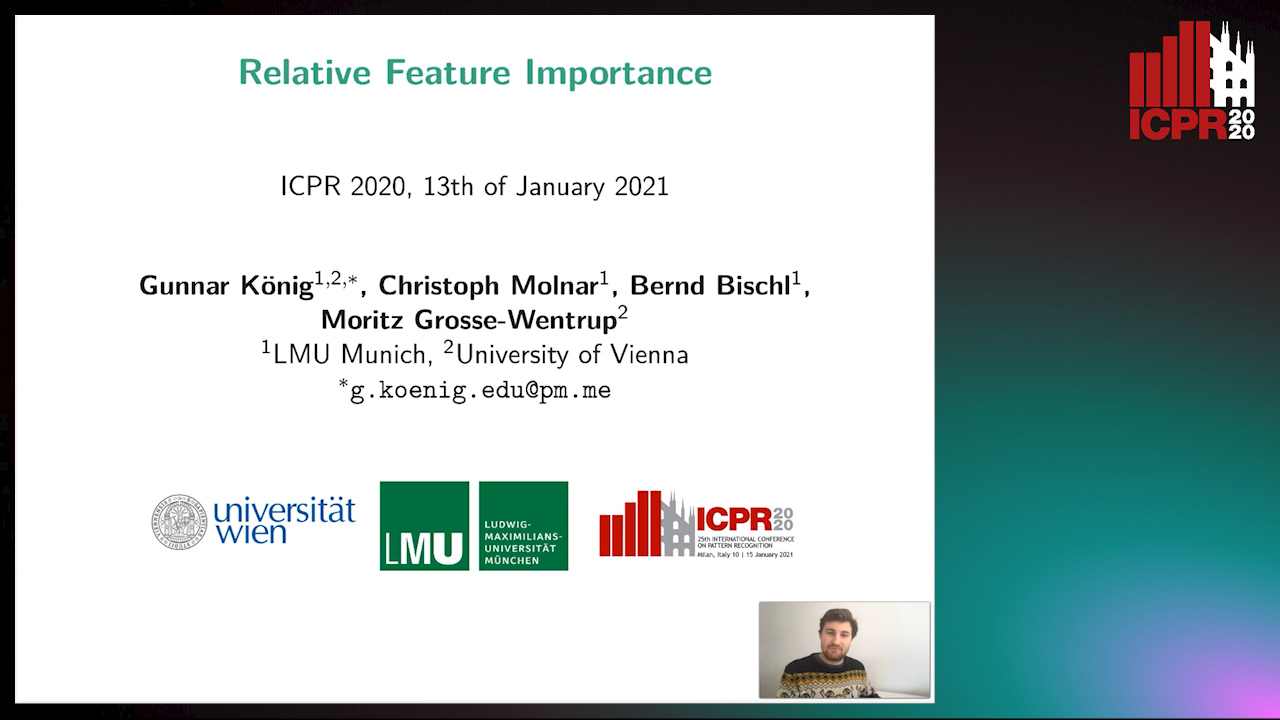
Auto-TLDR; Relative Feature Importance for Interpretable Machine Learning
Interpretable Machine Learning (IML) methods are used to gain insight into the relevance of a feature of interest for the performance of a model. Commonly used IML methods differ in whether they consider features of interest in isolation, e.g., Permutation Feature Importance (PFI), or in relation to all remaining feature variables, e.g., Conditional Feature Importance (CFI). As such, the perturbation mechanisms inherent to PFI and CFI represent extreme reference points. We introduce Relative Feature Importance (RFI), a generalization of PFI and CFI that allows for a more nuanced feature importance computation beyond the PFI versus CFI dichotomy. With RFI, the importance of a feature relative to any other subset of features can be assessed, including variables that were not available at training time. We derive general interpretation rules for RFI based on a detailed theoretical analysis of the implications of relative feature relevance, and demonstrate the method's usefulness on simulated examples.