Xiaohua Xiao
Papers from this author
Longitudinal Feature Selection and Feature Learning for Parkinson’s Disease Diagnosis and Prediction
Haijun Lei, Zhongwei Huang, Xiaohua Xiao, Yi Lei, En-Leng Tan, Baiying Lei, Shiqi Li
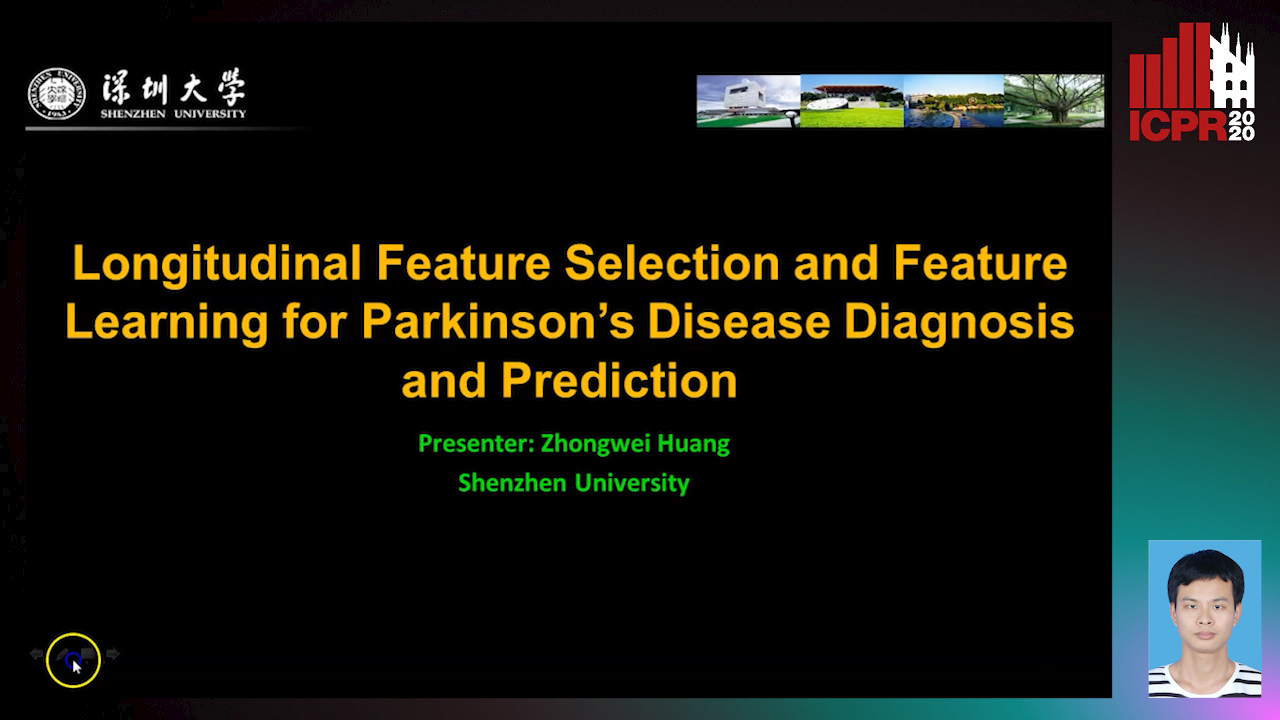
Auto-TLDR; Joint Learning from Multiple Modalities and Relations for Joint Disease Diagnosis and Prediction in Parkinson's Disease
Abstract Slides Poster Similar