Dmitry Sidnev
Paper download is intended for registered attendees only, and is
subjected to the IEEE Copyright Policy. Any other use is strongly forbidden.
Papers from this author
Building Computationally Efficient and Well-Generalizing Person Re-Identification Models with Metric Learning
Vladislav Sovrasov, Dmitry Sidnev
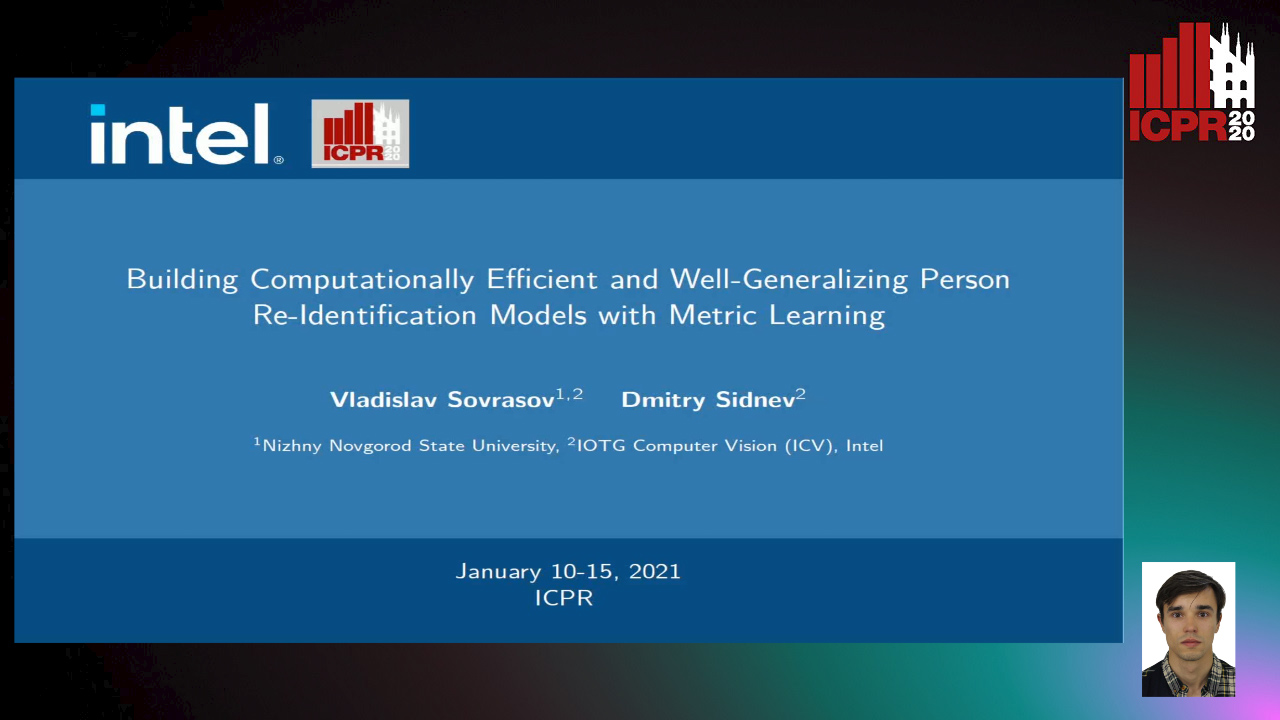
Auto-TLDR; Cross-Domain Generalization in Person Re-identification using Omni-Scale Network
This work considers the problem of domain shift in person re-identification.Being trained on one dataset, a re-identification model usually performs much worse on unseen data. Partially this gap is caused by the relatively small scale of person re-identification datasets (compared to face recognition ones, for instance), but it is also related to training objectives. We propose to use the metric learning objective, namely AM-Softmax loss, and some additional training practices to build well-generalizing, yet, computationally efficient models. We use recently proposed Omni-Scale Network (OSNet) architecture combined with several training tricks and architecture adjustments to obtain state-of-the art results in cross-domain generalization problem on a large-scale MSMT17 dataset in three setups: MSMT17-all->DukeMTMC, MSMT17-train->Market1501 and MSMT17-all->Market1501.