Théo Voillemin
Paper download is intended for registered attendees only, and is
subjected to the IEEE Copyright Policy. Any other use is strongly forbidden.
Papers from this author
2D Deep Video Capsule Network with Temporal Shift for Action Recognition
Théo Voillemin, Hazem Wannous, Jean-Philippe Vandeborre
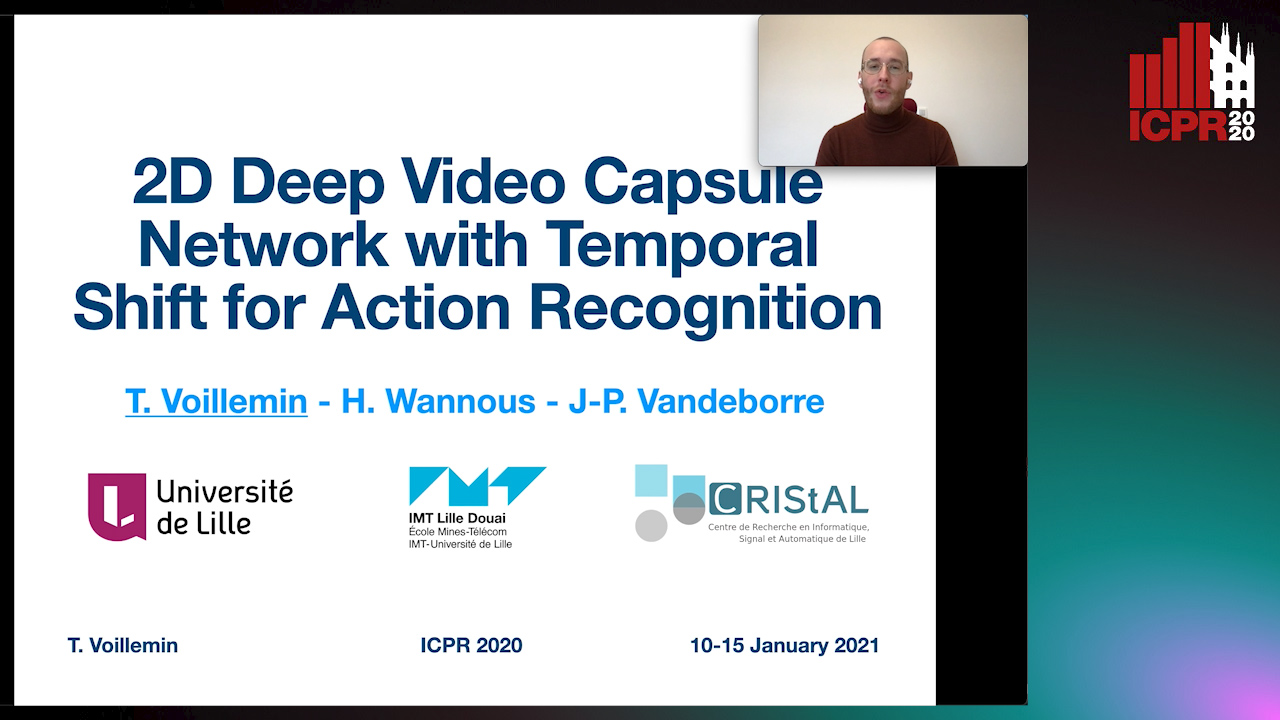
Auto-TLDR; Temporal Shift Module over Capsule Network for Action Recognition in Continuous Videos
Action recognition in continuous video streams is a growing field since the past few years. Deep learning techniques and in particular Convolutional Neural Networks (CNNs) achieved good results in this topic. However, intrinsic CNNs limitations begin to cap the results since 2D CNN cannot capture temporal information and 3D CNN are to much resource demanding for real-time applications. Capsule Network, evolution of CNN, already proves its interesting benefits on small and low informational datasets like MNIST but yet its true potential has not emerged. In this paper we tackle the action recognition problem by proposing a new architecture combining Temporal Shift module over deep Capsule Network. Temporal Shift module permits us to insert temporal information over 2D Capsule Network with a zero computational cost to conserve the lightness of 2D capsules and their ability to connect spatial features. Our proposed approach outperforms or brings near state-of-the-art results on color and depth information on public datasets like First Person Hand Action and DHG 14/28 with a number of parameters 10 to 40 times less than existing approaches.