Fangyu Wu
Paper download is intended for registered attendees only, and is
subjected to the IEEE Copyright Policy. Any other use is strongly forbidden.
Papers from this author
Pose-Robust Face Recognition by Deep Meta Capsule Network-Based Equivariant Embedding
Fangyu Wu, Jeremy Simon Smith, Wenjin Lu, Bailing Zhang
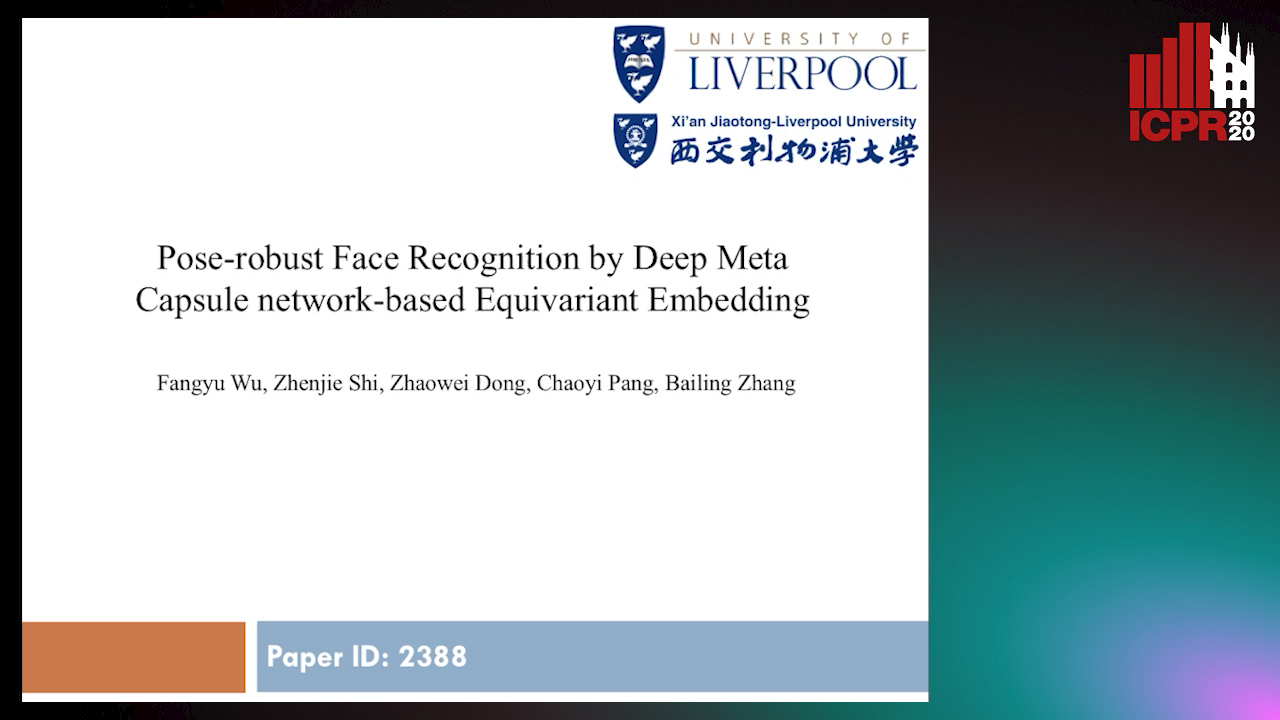
Auto-TLDR; Deep Meta Capsule Network-based Equivariant Embedding Model for Pose-Robust Face Recognition
Despite the exceptional success in face recognition related technologies, handling large pose variations still remains a key challenge. Current techniques for pose-robust face recognition either, directly extract pose-invariant features, or first synthesize a face that matches the target pose before feature extraction. It is more desirable to learn face representations equivariant to pose variations. To this end, this paper proposes a deep meta Capsule network-based Equivariant Embedding Model (DM-CEEM) with three distinct novelties. First, the proposed RB-CapsNet allows DM-CEEM to learn an equivariant embedding for pose variations and achieve the desired transformation for input face images. Second, we introduce a new version of a Capsule network called RB-CapsNet to extend CapsNet to perform a profile-to-frontal face transformation in deep feature space. Third, we train the DM-CEEM in a meta way by treating a single overall classification target as multiple sub-tasks that satisfy certain unknown probabilities. In each sub-task, we sample the support and query sets randomly. The experimental results on both controlled and in-the-wild databases demonstrate the superiority of DM-CEEM over state-of-the-art.