Yuki Inoue
Paper download is intended for registered attendees only, and is
subjected to the IEEE Copyright Policy. Any other use is strongly forbidden.
Papers from this author
Crack Detection As a Weakly-Supervised Problem: Towards Achieving Less Annotation-Intensive Crack Detectors
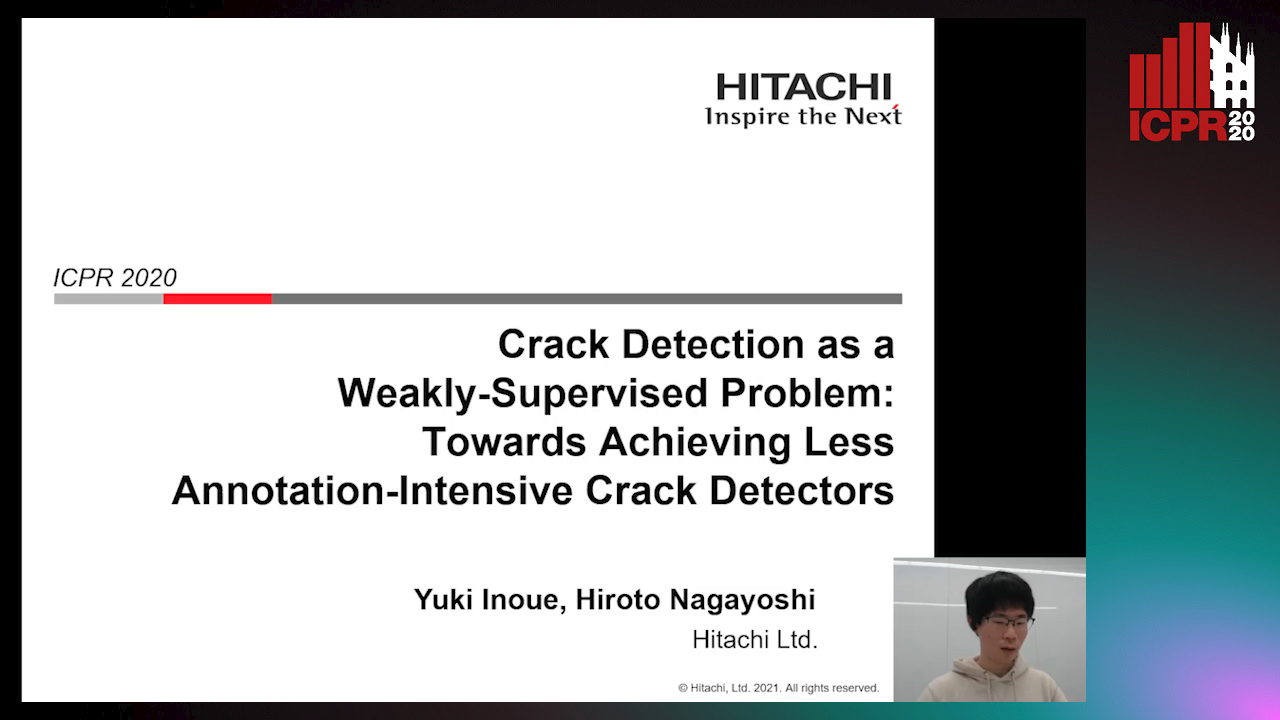
Auto-TLDR; A Weakly-supervised Framework for Automatic Crack Detection
Abstract Slides Poster Similar
Automatic crack detection is a critical task that has the potential to drastically reduce labor-intensive building and road inspections currently being done manually. Recent studies in this field have made significant improvements on detection accuracy. However, they often require costly annotation processes. In addition, to handle a wide variety of target domains, new batches of annotations are usually required for each new environment. This makes the data annotation cost a significant bottleneck when deploying crack detection systems. To resolve this issue, we formulate the crack detection problem as a weakly-supervised problem and propose a two-branched framework. By combining predictions from a supervised model trained with low quality annotations and predictions from pixel intensities, our framework is less affected by annotation quality. Experimental results show that the proposed framework retains a high accuracy even when provided with lower quality annotations.