Li Song
Paper download is intended for registered attendees only, and is
subjected to the IEEE Copyright Policy. Any other use is strongly forbidden.
Papers from this author
MBD-GAN: Model-Based Image Deblurring with a Generative Adversarial Network
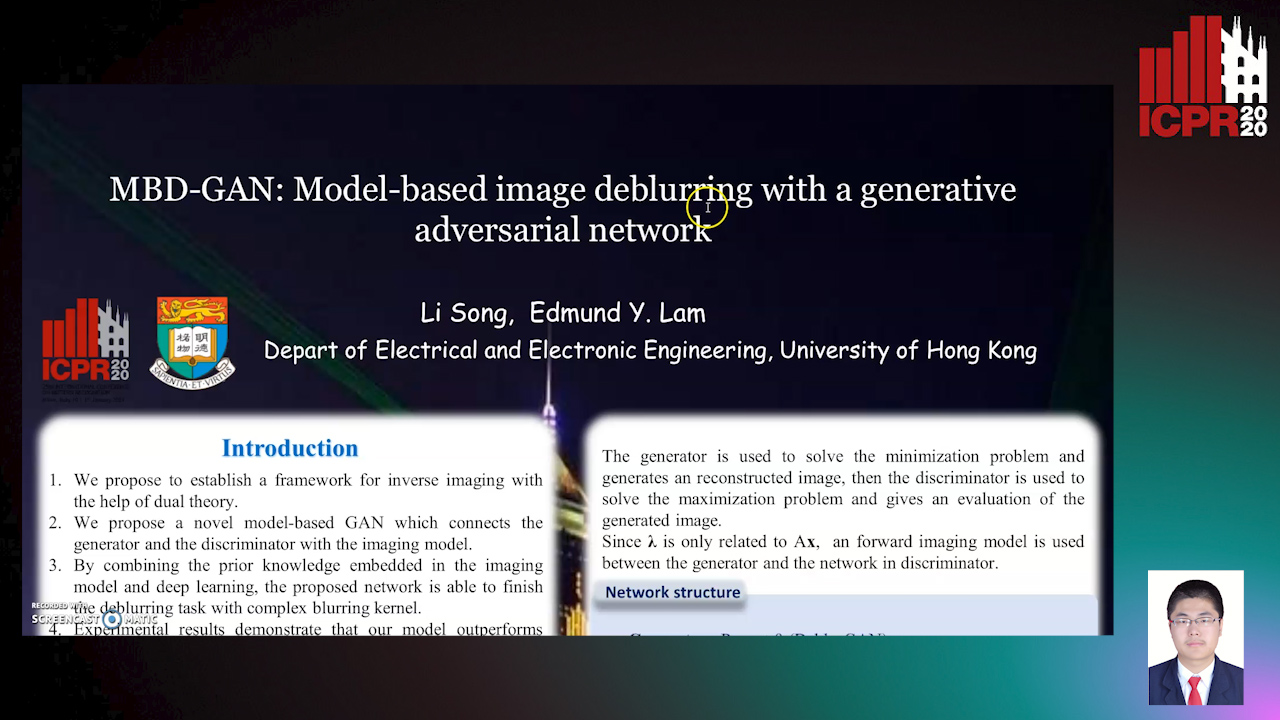
Auto-TLDR; Model-Based Deblurring GAN for Inverse Imaging
Abstract Slides Poster Similar
This paper presents a methodology to tackle inverse imaging problems by leveraging the synergistic power of imaging model and deep learning. The premise is that while learning-based techniques have quickly become the methods of choice in various applications, they often ignore the prior knowledge embedded in imaging models. Incorporating the latter has the potential to improve the image estimation. Specifically, we first provide a mathematical basis of using generative adversarial network (GAN) in inverse imaging through considering an optimization framework. Then, we develop the specific architecture that connects the generator and discriminator networks with the imaging model. While this technique can be applied to a variety of problems, from image reconstruction to super-resolution, we take image deblurring as the example here, where we show in detail the implementation and experimental results of what we call the model-based deblurring GAN (MBD-GAN).