Álvaro Casado Coscollá
Paper download is intended for registered attendees only, and is
subjected to the IEEE Copyright Policy. Any other use is strongly forbidden.
Papers from this author
RISEdb: A Novel Indoor Localization Dataset
Carlos Sanchez Belenguer, Erik Wolfart, Álvaro Casado Coscollá, Vitor Sequeira
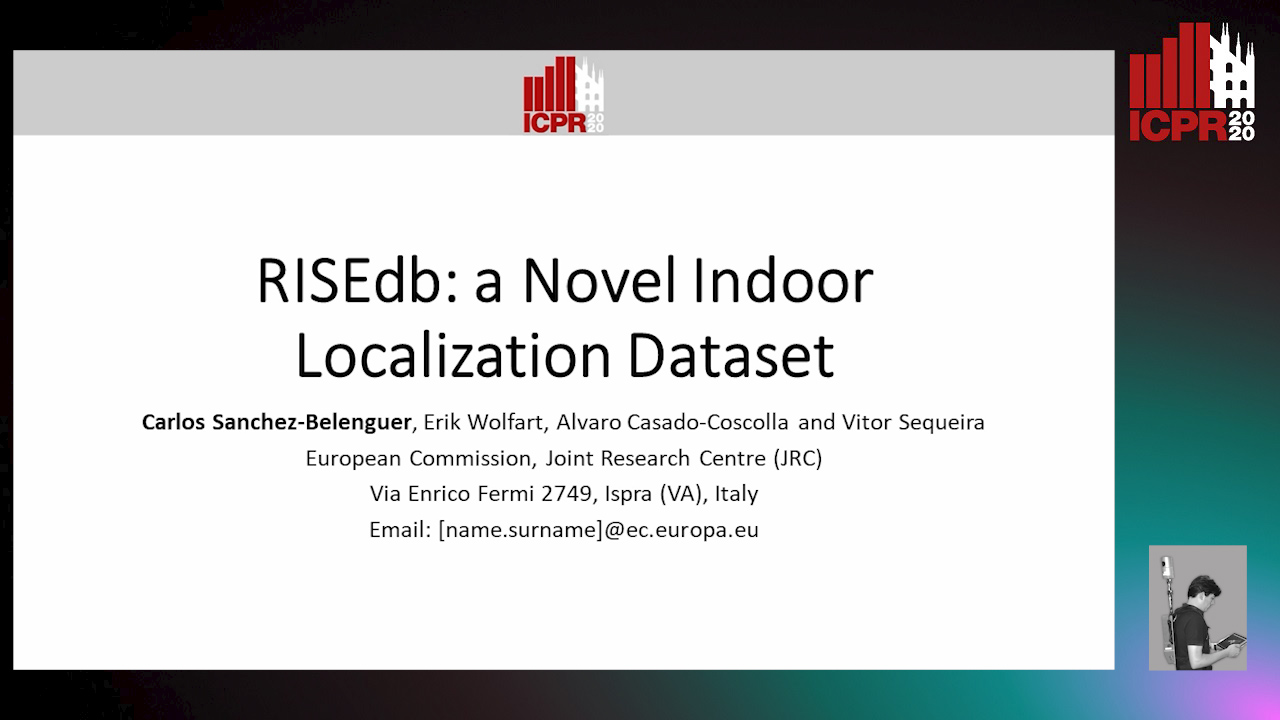
Auto-TLDR; Indoor Localization Using LiDAR SLAM and Smartphones: A Benchmarking Dataset
Abstract Slides Poster Similar
In this paper we introduce a novel public dataset for developing and benchmarking indoor localization systems. We have selected and 3D mapped a set of representative indoor environments including a large office building, a conference room, a workshop, an exhibition area and a restaurant. Our acquisition pipeline is based on a portable LiDAR SLAM backpack to map the buildings and to accurately track the pose of the user as it moves freely inside them. We introduce the calibration procedures that enable us to acquire and geo-reference live data coming from different independent sensors rigidly attached to the backpack. This has allowed us to collect long sequences of spherical and stereo images, together with all the sensor readings coming from a consumer smartphone and locate them inside the map with centimetre accuracy. The dataset addresses many of the limitations of existing indoor localization datasets regarding the scale and diversity of the mapped buildings; the number of acquired sequences under varying conditions; the accuracy of the ground-truth trajectory; the availability of a detailed 3D model and the availability of different sensor types. It enables the benchmarking of existing and the development of new indoor localization approaches, in particular for deep learning based systems that require large amounts of labeled training data.