Jani Boutellier
Paper download is intended for registered attendees only, and is
subjected to the IEEE Copyright Policy. Any other use is strongly forbidden.
Papers from this author
Can You Trust Your Pose? Confidence Estimation in Visual Localization
Luca Ferranti, Xiaotian Li, Jani Boutellier, Juho Kannala
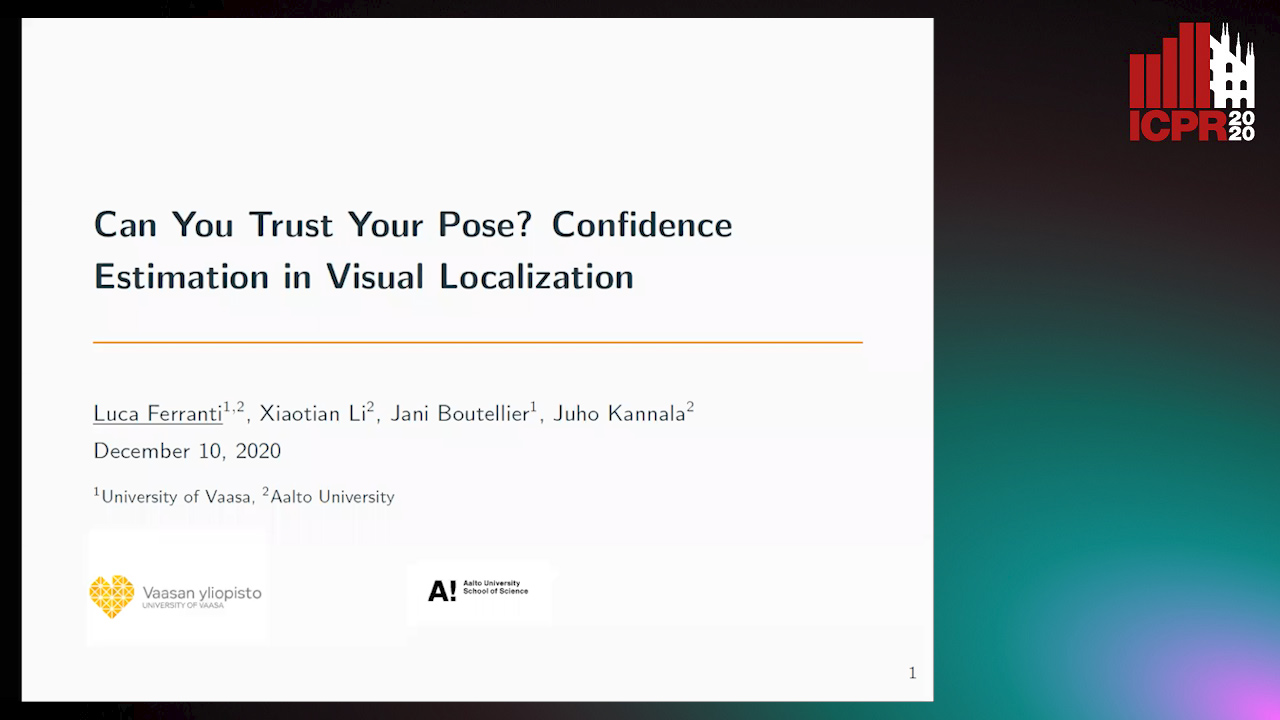
Auto-TLDR; Pose Confidence Estimation in Large-Scale Environments: A Light-weight Approach to Improving Pose Estimation Pipeline
Abstract Slides Poster Similar
Camera pose estimation in large-scale environments is still an open question and, despite recent promising results, it may still fail in some situations. The research so far has focused on improving subcomponents of estimation pipelines, to achieve more accurate poses. However, there is no guarantee for the result to be correct, even though the correctness of pose estimation is critically important in several visual localization applications, such as in autonomous navigation. In this paper we bring to attention a novel research question, pose confidence estimation, where we aim at quantifying how reliable the visually estimated pose is. We develop a novel confidence measure to fulfill this task and show that it can be flexibly applied to different datasets, indoor or outdoor, and for various visual localization pipelines. We also show that the proposed techniques can be used to accomplish a secondary goal: improving the accuracy of existing pose estimation pipelines. Finally, the proposed approach is computationally light-weight and adds only a negligible increase to the computational effort of pose estimation.