Jingyi Song
Paper download is intended for registered attendees only, and is
subjected to the IEEE Copyright Policy. Any other use is strongly forbidden.
Papers from this author
Construction Worker Hardhat-Wearing Detection Based on an Improved BiFPN
Chenyang Zhang, Zhiqiang Tian, Jingyi Song, Yaoyue Zheng, Bo Xu
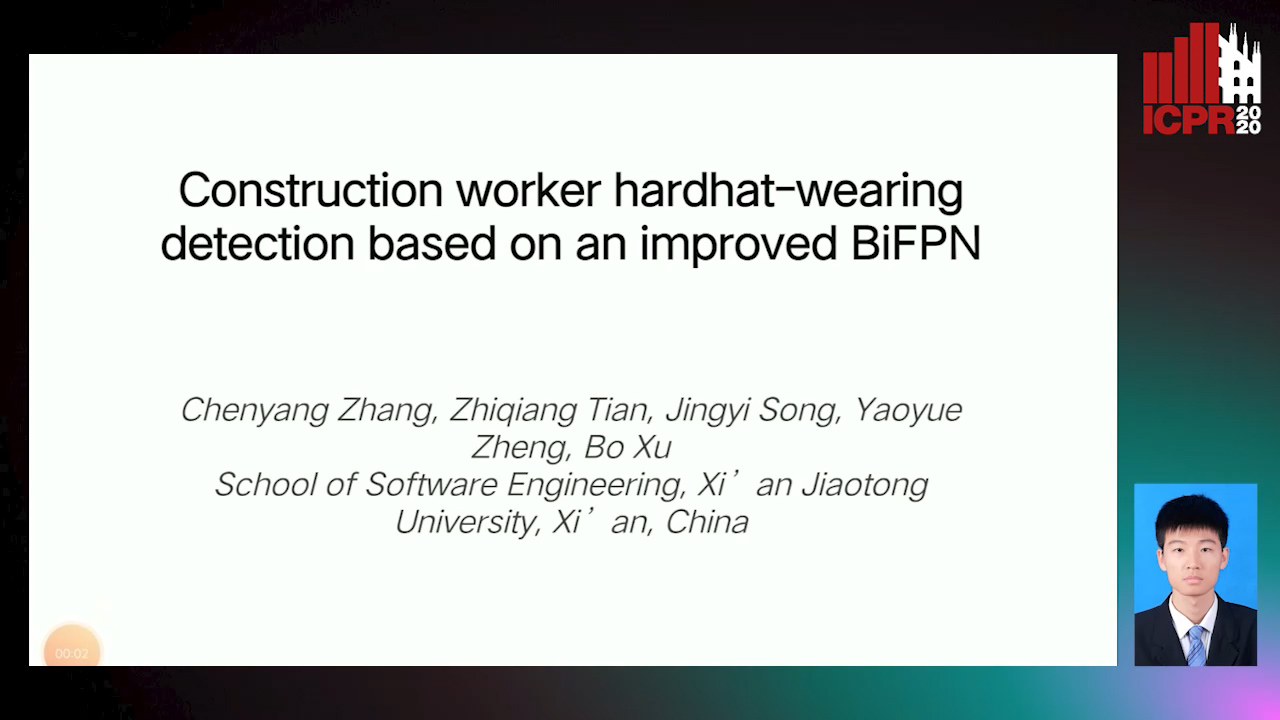
Auto-TLDR; A One-Stage Object Detection Method for Hardhat-Wearing in Construction Site
Abstract Slides Poster Similar
Work in the construction site is considered to be one of the occupations with the highest safety risk factor. Therefore, safety plays an important role in construction site. One of the most fundamental safety rules in construction site is to wear a hardhat. To strengthen the safety of the construction site, most of the current methods use multi-stage method for hardhat-wearing detection. These methods have limitations in terms of adaptability and generalizability. In this paper, we propose a one-stage object detection method based on convolutional neural network. We present a multi-scale strategy that selects the high-resolution feature maps of DarkNet-53 to effectively identify small-scale hardhats. In addition, we propose an improved weighted bi-directional feature pyramid network (BiFPN), which could fuse more semantic features from more scales. The proposed method can not only detect hardhat-wearing, but also identify the color of the hardhat. Experimental results show that the proposed method achieves a mAP of 87.04%, which outperforms several state-of-the-art methods on a public dataset.