Mengyuan Ding
Paper download is intended for registered attendees only, and is
subjected to the IEEE Copyright Policy. Any other use is strongly forbidden.
Papers from this author
Learning a Dynamic High-Resolution Network for Multi-Scale Pedestrian Detection
Mengyuan Ding, Shanshan Zhang, Jian Yang
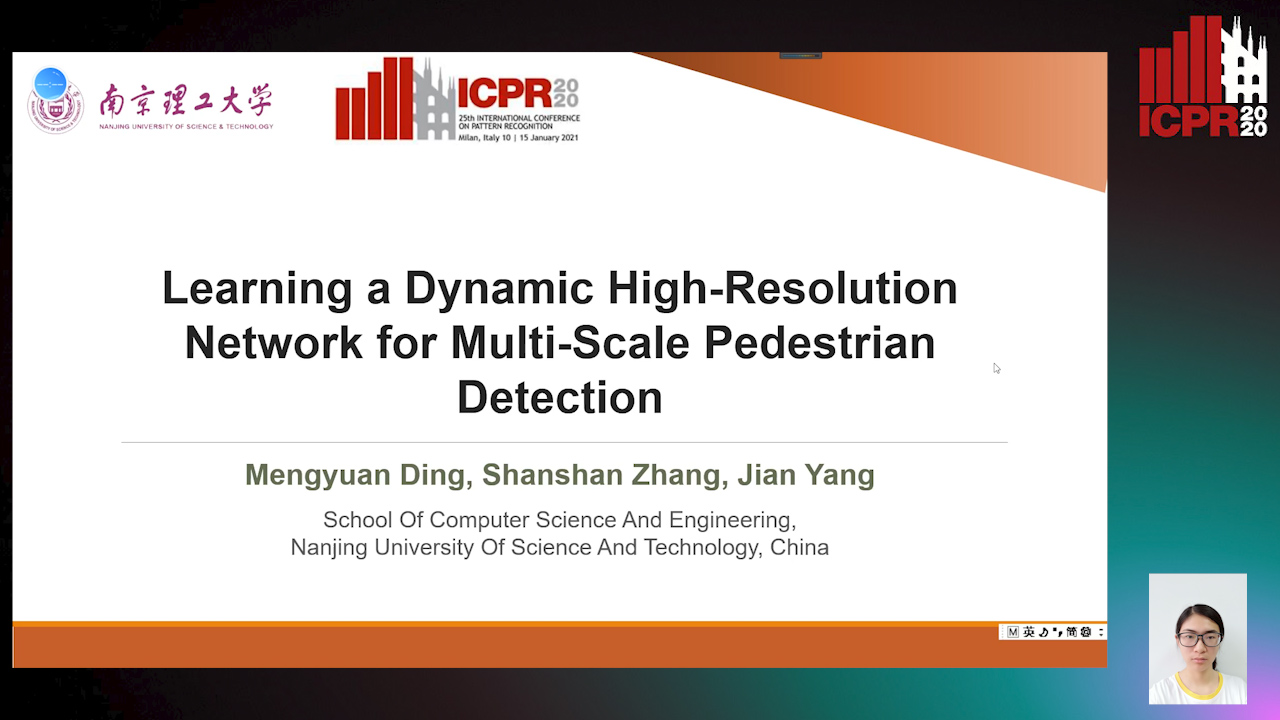
Auto-TLDR; Learningable Dynamic HRNet for Pedestrian Detection
Abstract Slides Poster Similar
Pedestrian detection is a canonical instance of object detection in computer vision. In practice, scale variation is one of the key challenges, resulting in unbalanced performance across different scales. Recently, the High-Resolution Network (HRNet) has become popular because high-resolution feature representations are more friendly to small objects. However, when we apply HRNet for pedestrian detection, we observe that it improves for small pedestrians on one hand, but hurts the performance for larger ones on the other hand. To overcome this problem, we propose a learnable Dynamic HRNet (DHRNet) aiming to generate different network paths adaptive to different scales. Specifically, we construct a parallel multi-branch architecture and add a soft conditional gate module allowing for dynamic feature fusion. Both branches share all the same parameters except the soft gate module. Experimental results on CityPersons and Caltech benchmarks indicate that our proposed dynamic HRNet is more capable of dealing with pedestrians of various scales, and thus improves the performance across different scales consistently.