Mingyue Feng
Paper download is intended for registered attendees only, and is
subjected to the IEEE Copyright Policy. Any other use is strongly forbidden.
Papers from this author
Improving Low-Resolution Image Classification by Super-Resolution with Enhancing High-Frequency Content
Liguo Zhou, Guang Chen, Mingyue Feng, Alois Knoll
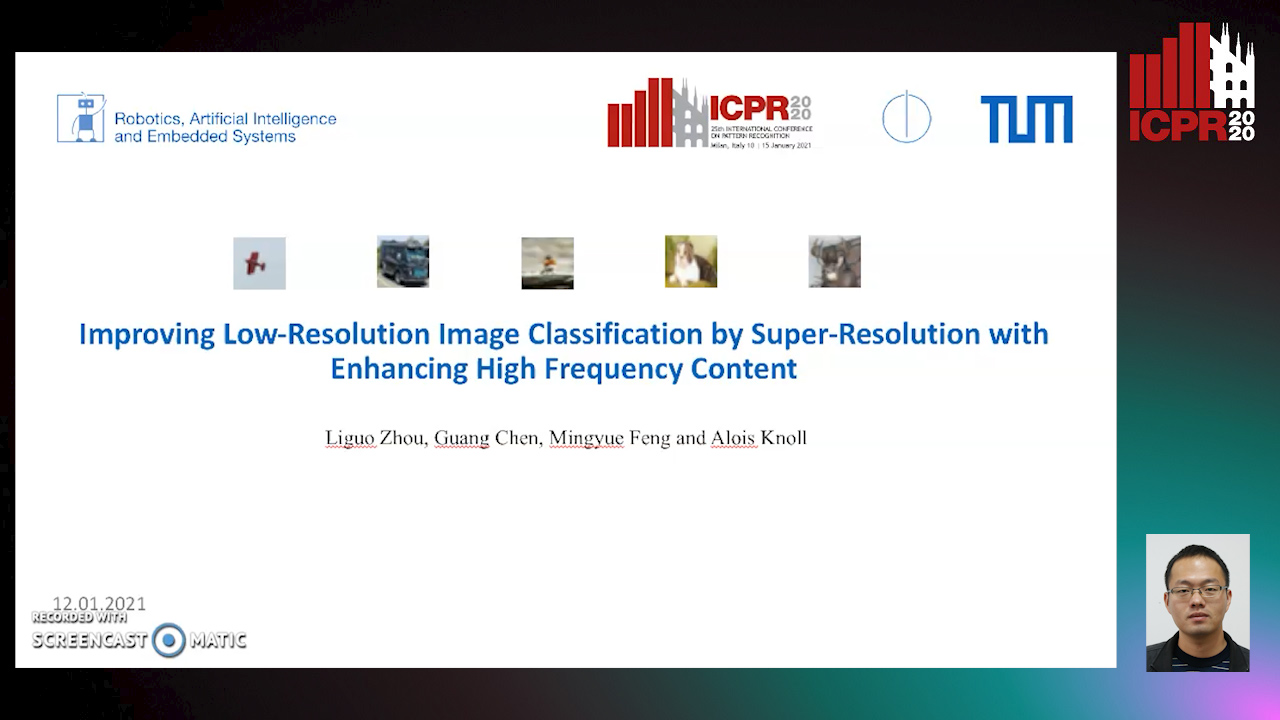
Auto-TLDR; Super-resolution for Low-Resolution Image Classification
Abstract Slides Poster Similar
With the prosperous development of Convolutional Neural Networks, currently they can perform excellently on visual understanding tasks when the input images are high quality and common quality images. However, large degradation in performance always occur when the input images are low quality images. In this paper, we propose a new super-resolution method in order to improve the classification performance for low-resolution images. In an image, the regions in which pixel values vary dramatically contain more abundant high frequency contents compared to other parts. Based on this fact, we design a weight map and integrate it with a super-resolution CNN training framework. During the process of training, this weight map can find out positions of the high frequency pixels in ground truth high-resolution images. After that, the pixel-level loss function takes effect only at these found positions to minimize the difference between reconstructed high-resolution images and ground truth high-resolution images. Compared with other state-of-the-art super-resolution methods, the experiment results show that our method can recover more high-frequency contents in high-resolution image reconstructing, and better improve the classification accuracy after low-resolution image preprocessing.