Hao Dou
Paper download is intended for registered attendees only, and is
subjected to the IEEE Copyright Policy. Any other use is strongly forbidden.
Papers from this author
Deep Top-Rank Counter Metric for Person Re-Identification
Chen Chen, Hao Dou, Xiyuan Hu, Silong Peng
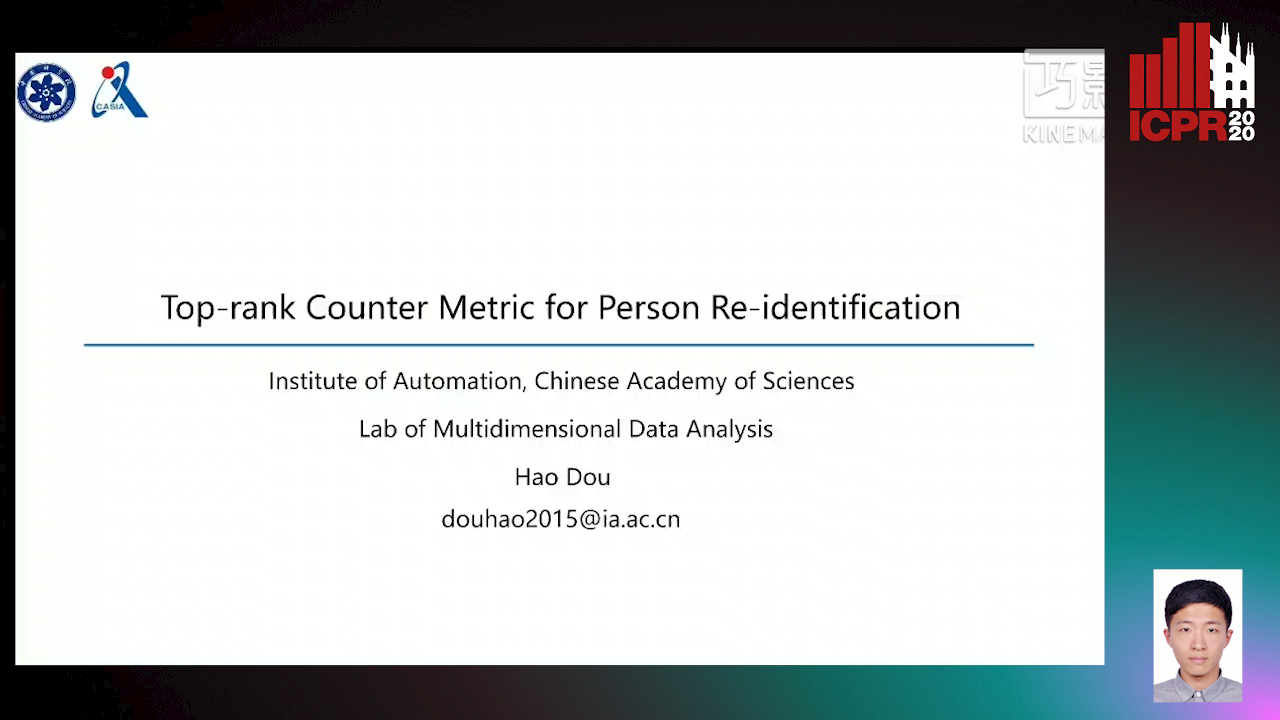
Auto-TLDR; Deep Top-Rank Counter Metric for Person Re-identification
Abstract Slides Poster Similar
In the research field of person re-identification, deep metric learning that guides the efficient and effective embedding learning serves as one of the most fundamental tasks. Recent efforts of the loss function based deep metric learning methods mainly focus on the top rank accuracy optimization by minimiz- ing the distance difference between the correctly matching sample pair and wrongly matched sample pair. However, it is more straightforward to count the occurrences of correct top-rank candidates and maximize the counting results for better top rank accuracy. In this paper, we propose a generalized logistic function based metric with effective practicalness in deep learning, namely the“deep top-rank counter metric”, to approximately optimize the counted occurrences of the correct top-rank matches. The properties that qualify the proposed metric as a well-suited deep re-identification metric have been discussed and a progressive hard sample mining strategy is also introduced for effective training and performance boosting. The extensive experiments show that the proposed top-rank counter metric outperforms other loss function based deep metrics and achieves the state-of- the-art accuracies.