Xiaogang Jia
Paper download is intended for registered attendees only, and is
subjected to the IEEE Copyright Policy. Any other use is strongly forbidden.
Papers from this author
CenterRepp: Predict Central Representative Point Set's Distribution for Detection
Yulin He, Limeng Zhang, Wei Chen, Xin Luo, Chen Li, Xiaogang Jia
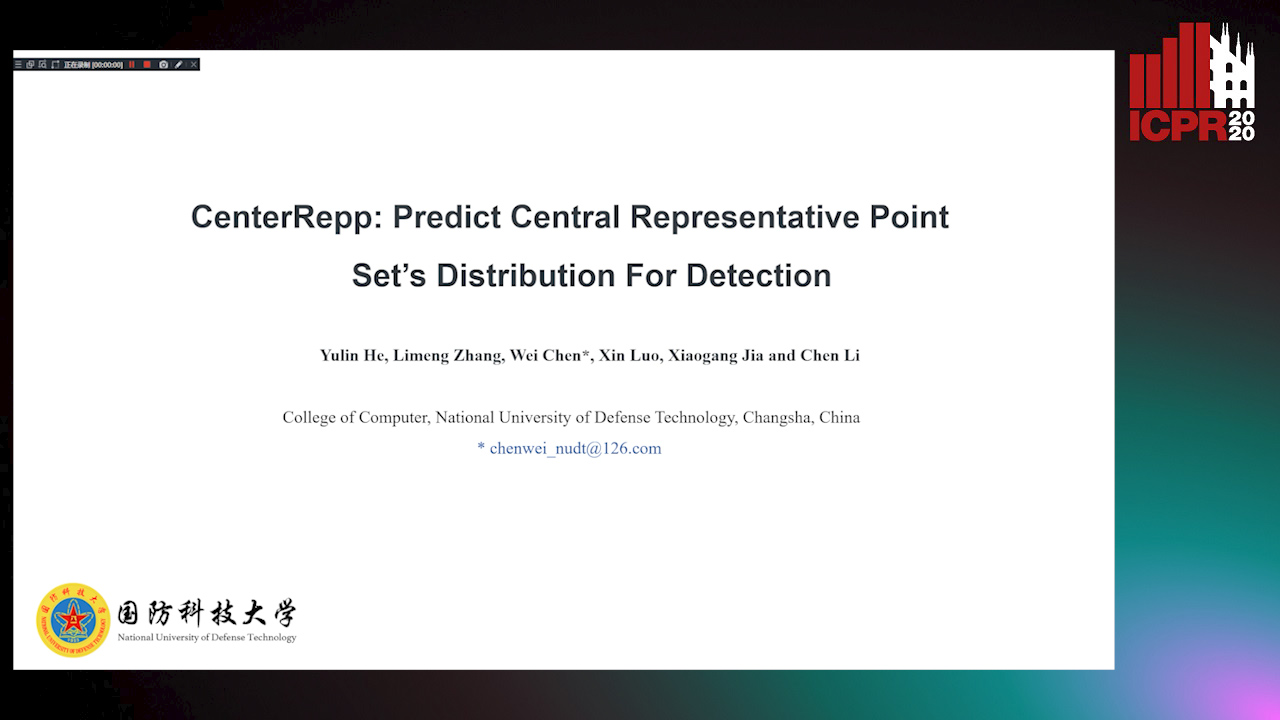
Auto-TLDR; CRPDet: CenterRepp Detector for Object Detection
Abstract Slides Poster Similar
Object detection has long been an important issue in the discipline of scene understanding. Existing researches mainly focus on the object itself, ignoring its surrounding environment. In fact, the surrounding environment provides abundant information to help detectors classify and locate objects. This paper proposes CRPDet, viz. CenterRepp Detector, a framework for object detection. The main function of CRPDet is accomplished by the CenterRepp module, which takes into account the surrounding environment by predicting the distribution of the central representative points. CenterRepp converts labeled object frames into the mean and standard variance of the sampling points’ distribution. This helps increase the receptive field of objects, breaking the limitation of object frames. CenterRepp defines a position-fixed center point with significant weights, avoiding to sample all points in the surroundings. Experiments on the COCO test-dev detection benchmark demonstrates that our proposed CRPDet has comparable performance with state-of-the-art detectors, achieving 39.4 mAP with 51 FPS tested under single size input.