Yao Yao
Paper download is intended for registered attendees only, and is
subjected to the IEEE Copyright Policy. Any other use is strongly forbidden.
Papers from this author
Learning Stereo Matchability in Disparity Regression Networks
Jingyang Zhang, Yao Yao, Zixin Luo, Shiwei Li, Tianwei Shen, Tian Fang, Long Quan
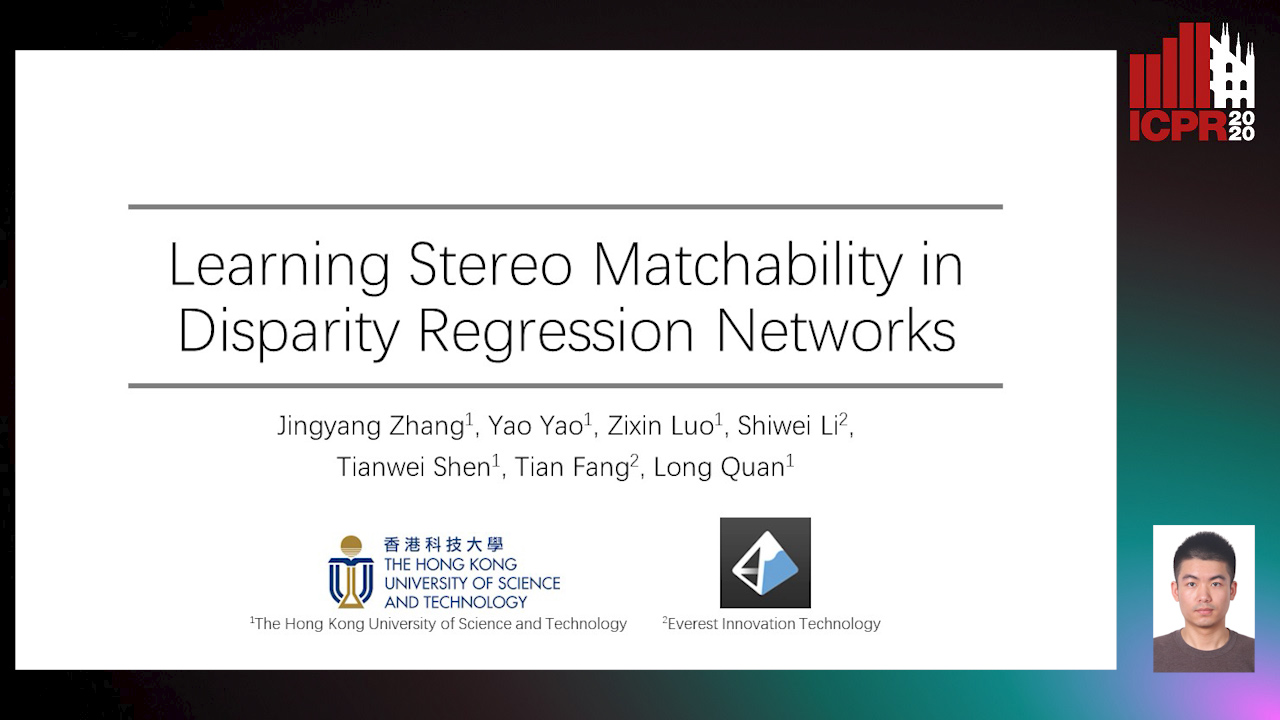
Auto-TLDR; Deep Stereo Matchability for Weakly Matchable Regions
Learning-based stereo matching has recently achieved promising results, yet still suffers difficulties in establishing reliable matches in weakly matchable regions that are textureless, non-Lambertian, or occluded. In this paper, we address this challenge by proposing a stereo matching network that considers pixel-wise matchability. Specifically, the network jointly regresses disparity and matchability maps from 3D probability volume through expectation and entropy operations. Next, a learned attenuation is applied as the robust loss function to alleviate the influence of weakly matchable pixels in the training. Finally, a matchability-aware disparity refinement is introduced to improve the depth inference in weakly matchable regions. The proposed deep stereo matchability (DSM) framework can improve the matching result or accelerate the computation while still guaranteeing the quality. Moreover, the DSM framework is portable to many recent stereo networks. Extensive experiments are conducted on Scene Flow and KITTI stereo datasets to demonstrate the effectiveness of the proposed framework over the state-of-the-art learning-based stereo methods.