Saleem Ahmed
Paper download is intended for registered attendees only, and is
subjected to the IEEE Copyright Policy. Any other use is strongly forbidden.
Papers from this author
Equation Attention Relationship Network (EARN) : A Geometric Deep Metric Framework for Learning Similar Math Expression Embedding
Saleem Ahmed, Kenny Davila, Srirangaraj Setlur, Venu Govindaraju
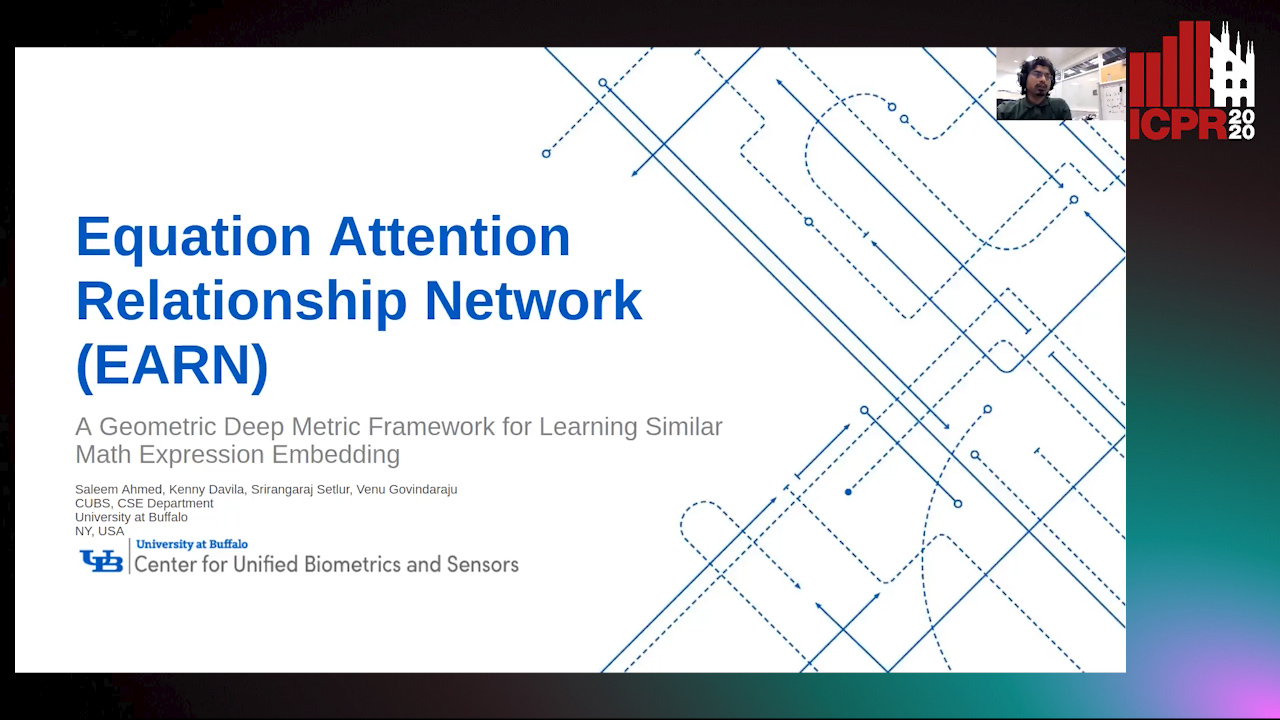
Auto-TLDR; Representational Learning for Similarity Based Retrieval of Mathematical Expressions
Abstract Slides Poster Similar
Representational Learning in the form of high dimensional embeddings have been used for multiple pattern recognition applications. There has been a significant interest in building embedding based systems for learning representationsin the mathematical domain. At the same time, retrieval of structured information such as mathematical expressions is an important need for modern IR systems. In this work, our motivation is to introduce a robust framework for learning representations for similarity based retrieval of mathematical expressions. Given a query by example, the embedding can find the closest matching expression as a function of euclidean distance between them. We leverage recent advancements in image-based and graph-based deep learning algorithms to learn our similarity embeddings. We do this first, by using uni-modal encoders in graph space and image space and then, a multi-modal combination of the same. To overcome the lack of training data, we force the networks to learn a deep metric using triplets generated with a heuristic scoring function. We also adopt a custom strategy for mining hard samples to train our neural networks. Our system produces rankings similar to those generated by the original scoring function, but using only a fraction of the time. Our results establish the viability of using such a multi-modal embedding for this task.