Hyunjae Lee
Paper download is intended for registered attendees only, and is
subjected to the IEEE Copyright Policy. Any other use is strongly forbidden.
Papers from this author
KoreALBERT: Pretraining a Lite BERT Model for Korean Language Understanding
Hyunjae Lee, Jaewoong Yun, Bongkyu Hwang, Seongho Joe, Seungjai Min, Youngjune Gwon
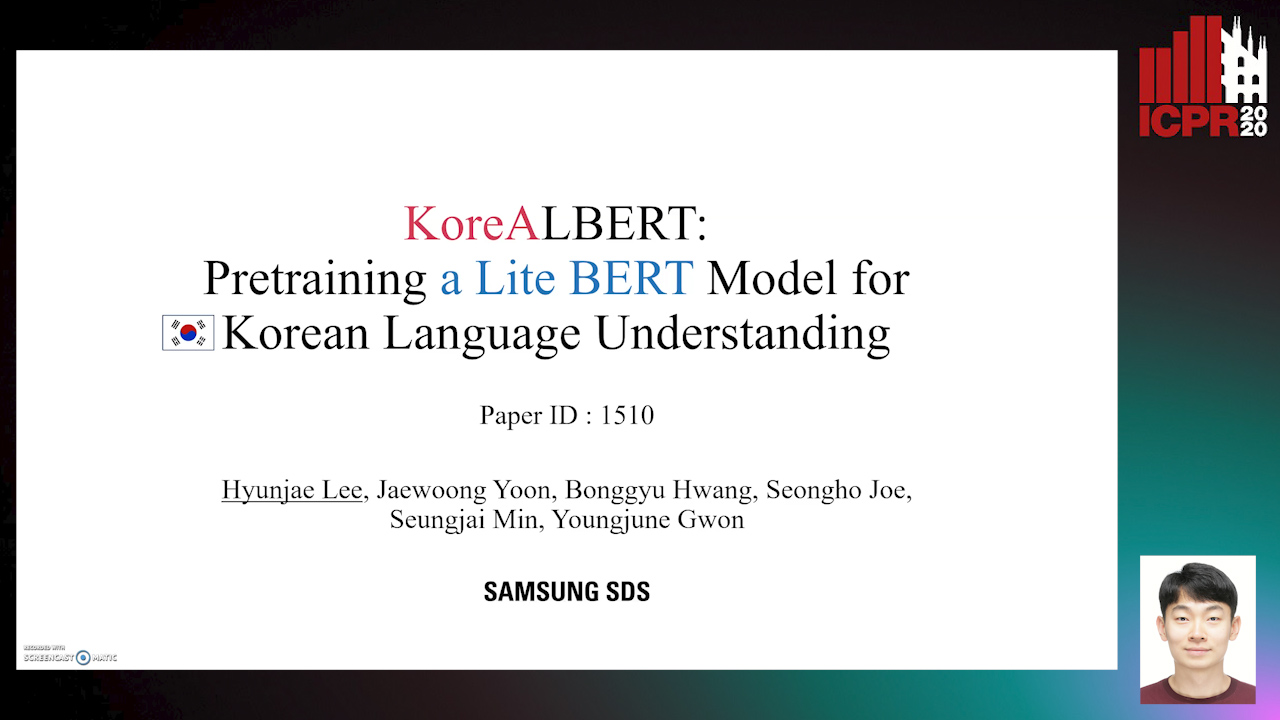
Auto-TLDR; KoreALBERT: A monolingual ALBERT model for Korean language understanding
Abstract Slides Poster Similar
Abstract—A Lite BERT (ALBERT) has been introduced to scale-up deep bidirectional representation learning for natural languages. Due to the lack of pretrained ALBERT models for Korean language, the best available practice is the multilingual model or resorting back to the any other BERT-based model. In this paper, we develop and pretrain KoreALBERT, a monolingual ALBERT model specifically for Korean language understanding. We introduce a new training objective, namely Word Order Prediction (WOP), and use alongside the existing MLM and SOP criteria to the same architecture and model parameters. Despite having significantly fewer model parameters (thus, quicker to train), our pretrained KoreALBERT outperforms its BERT counterpart on KorQuAD 1.0 benchmark for machine reading comprehension. Consistent with the empirical results in English by Lan et al., KoreALBERT seems to improve downstream task performance involving multi-sentence encoding for Korean language. The pretrained KoreALBERT is publicly available to encourage research and application development for Korean NLP.