Shuwei Wang
Paper download is intended for registered attendees only, and is
subjected to the IEEE Copyright Policy. Any other use is strongly forbidden.
Papers from this author
A Weak Coupling of Semi-Supervised Learning with Generative Adversarial Networks for Malware Classification
Shuwei Wang, Qiuyun Wang, Zhengwei Jiang, Xuren Wang, Rongqi Jing
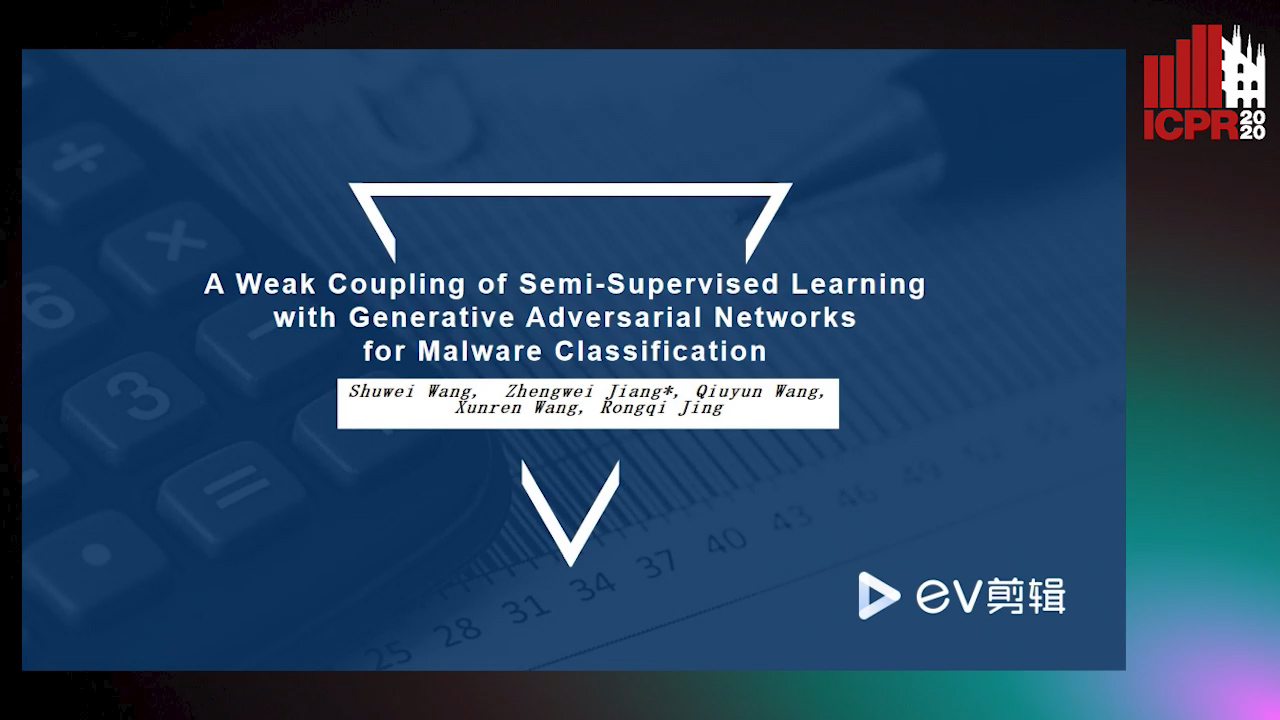
Auto-TLDR; IMIR: An Improved Malware Image Rescaling Algorithm Using Semi-supervised Generative Adversarial Network
Abstract Slides Poster Similar
Malware classification helps to understand its purpose and is also an important part of attack detection. And it is also an important part of discovering attacks. Due to continuous innovation and development of artificial intelligence, it is a trend to combine deep learning with malware classification. In this paper, we propose an improved malware image rescaling algorithm (IMIR) based on local mean algorithm. Its main goal of IMIR is to reduce the loss of information from samples during the process of converting binary files to image files. Therefore, we construct a neural network structure based on VGG model, which is suitable for image classification. In the real world, a mass of malware family labels are inaccurate or lacking. To deal with this situation, we propose a novel method to train the deep neural network by Semi-supervised Generative Adversarial Network (SGAN), which only needs a small amount of malware that have accurate labels about families. By integrating SGAN with weak coupling, we can retain the weak links of supervised part and unsupervised part of SGAN. It improves the accuracy of malware classification by making classifiers more independent of discriminators. The results of experimental demonstrate that our model achieves exhibiting favorable performance. The recalls of each family in our data set are all higher than 93.75%.