Keiichi Yamada
Paper download is intended for registered attendees only, and is
subjected to the IEEE Copyright Policy. Any other use is strongly forbidden.
Papers from this author
Few-Shot Learning Based on Metric Learning Using Class Augmentation
Susumu Matsumi, Keiichi Yamada
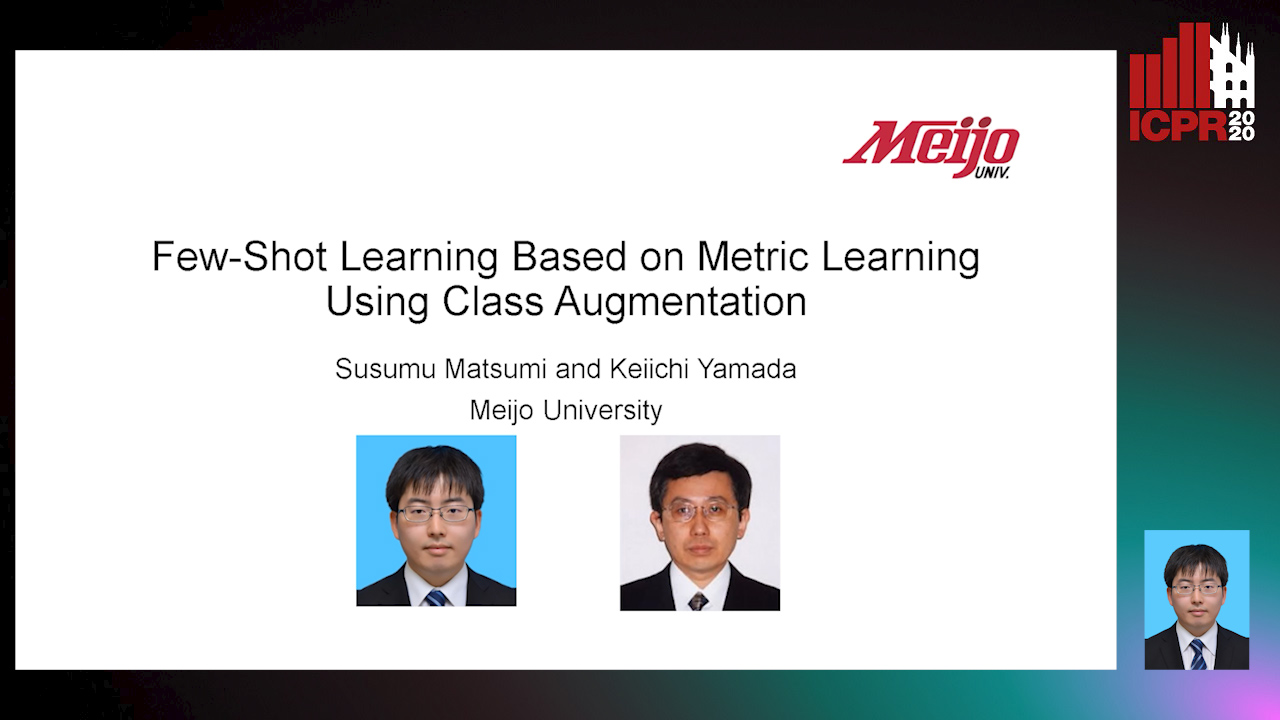
Auto-TLDR; Metric Learning for Few-shot Learning
Abstract Slides Poster Similar
Few-shot learning is a machine learning problem in which new categories are learned from only a few samples. One approach for few-shot learning is metric learning, which learns an embedding space in which learning is efficient for few-shot samples. In this paper, we focus on metric learning and demonstrate that the number of classes in the training data used for metric learning has a greater impact on the accuracy of few-shot learning than the number of samples per class. We propose a few-shot learning approach based on metric learning in which the number of classes in the training data for performing metric learning is increased. The number of classes is augmented by synthesizing samples of imaginary classes at a feature level from the original training data. The proposed method is evaluated on the miniImageNet dataset using the nearest neighbor method or a support vector machine as the classifier, and the effectiveness of the approach is demonstrated.