Shinichiro Omachi
Paper download is intended for registered attendees only, and is
subjected to the IEEE Copyright Policy. Any other use is strongly forbidden.
Papers from this author
Fidelity-Controllable Extreme Image Compression with Generative Adversarial Networks
Shoma Iwai, Tomo Miyazaki, Yoshihiro Sugaya, Shinichiro Omachi
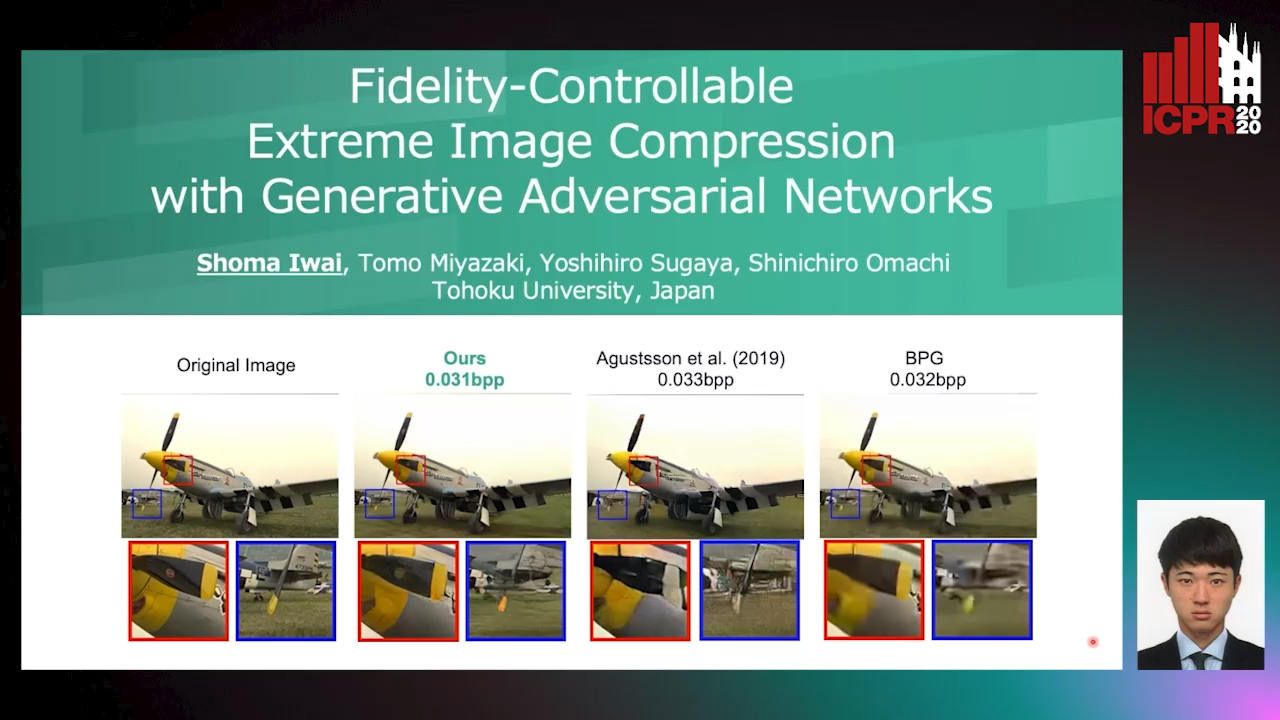
Auto-TLDR; GAN-based Image Compression at Low Bitrates
We propose a GAN-based image compression method working at extremely low bitrates below 0.1bpp. Most existing learned image compression methods suffer from blur at extremely low bitrates. Although GAN can help to reconstruct sharp images, there are two drawbacks. First, GAN makes train- ing unstable. Second, the reconstructions often contain unpleasing noise or artifacts. To address both of the drawbacks, our method adopts two-stage training and network interpolation. The two- stage training is effective to stabilize the training. Moreover, the network interpolation utilizes the models in both stages and reduces undesirable noise and artifacts, while maintaining important edges. Hence, we can control the trade-off between perceptual quality and fidelity without re-training models. The experimental results show that our model can reconstruct high quality images. Furthermore, our user study confirms that our reconstructions are preferable to state-of-the-art GAN-based image compression model.