Yipeng Deng
Paper download is intended for registered attendees only, and is
subjected to the IEEE Copyright Policy. Any other use is strongly forbidden.
Papers from this author
Selective Kernel and Motion-Emphasized Loss Based Attention-Guided Network for HDR Imaging of Dynamic Scenes
Yipeng Deng, Qin Liu, Takeshi Ikenaga
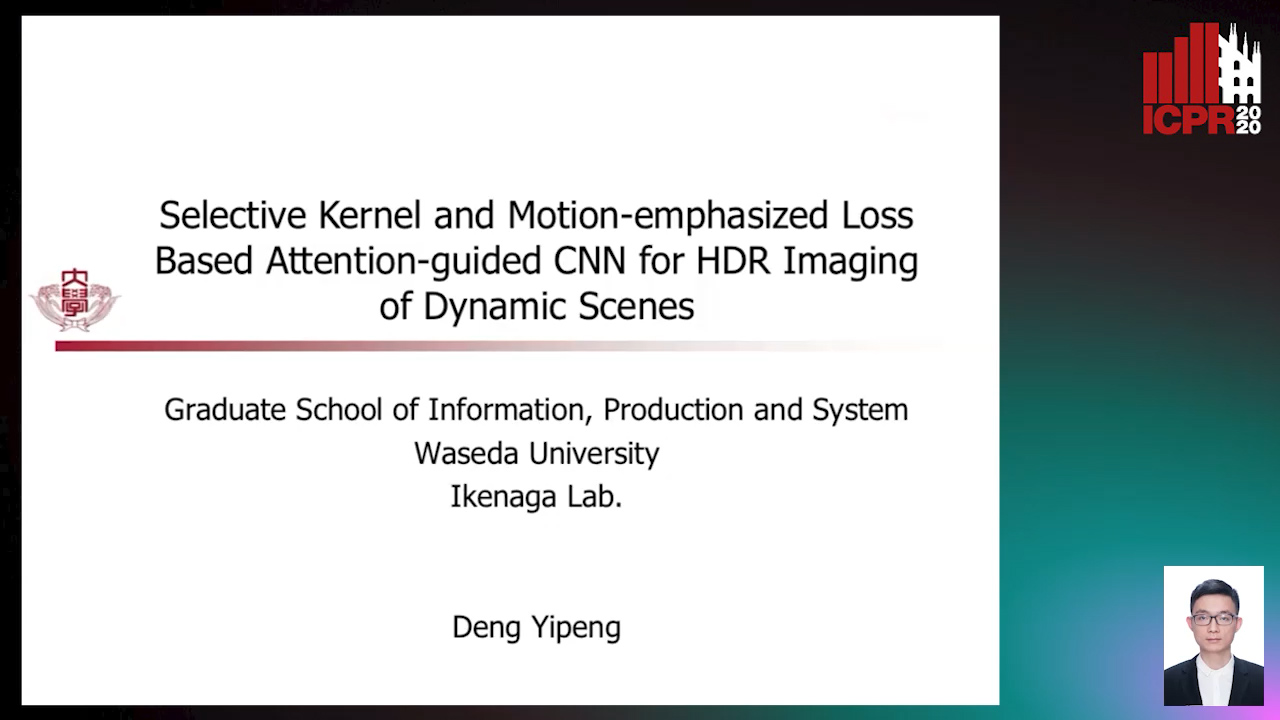
Auto-TLDR; SK-AHDRNet: A Deep Network with attention module and motion-emphasized loss function to produce ghost-free HDR images
Abstract Slides Poster Similar
Ghost-like artifacts caused by ill-exposed and motion areas is one of the most challenging problems in high dynamic range (HDR) image reconstruction.When the motion range is small, previous methods based on optical flow or patch-match can suppress ghost-like artifacts by first aligning input images before merging them.However, they are not robust enough and still produce artifacts for challenging scenes where large foreground motions exist.To this end, we propose a deep network with attention module and motion-emphasized loss function to produce ghost-free HDR images. In attention module, we use channel and spatial attention to guide network to emphasize important components such as motion and saturated areas automatically. With the purpose of being robust to images with different resolutions and objects with distinct scale, we adopt the selective kernel network as the basic framework for channel attention. In addition to the attention module, the motion-emphasized loss function based on the motion and ill-exposed areas mask is designed to help network reconstruct motion areas. Experiments on the public dataset indicate that the proposed SK-AHDRNet produces ghost-free results where detail in ill-exposed areas is well recovered. The proposed method scores 43.17 with PSNR metric and 61.02 with HDR-VDP-2 metric on test which outperforms all conventional works. According to quantitative and qualitative evaluations, the proposed method can achieve state-of-the-art performance.