Shao-Lun Huang
Paper download is intended for registered attendees only, and is
subjected to the IEEE Copyright Policy. Any other use is strongly forbidden.
Papers from this author
Person Recognition with HGR Maximal Correlation on Multimodal Data
Yihua Liang, Fei Ma, Yang Li, Shao-Lun Huang
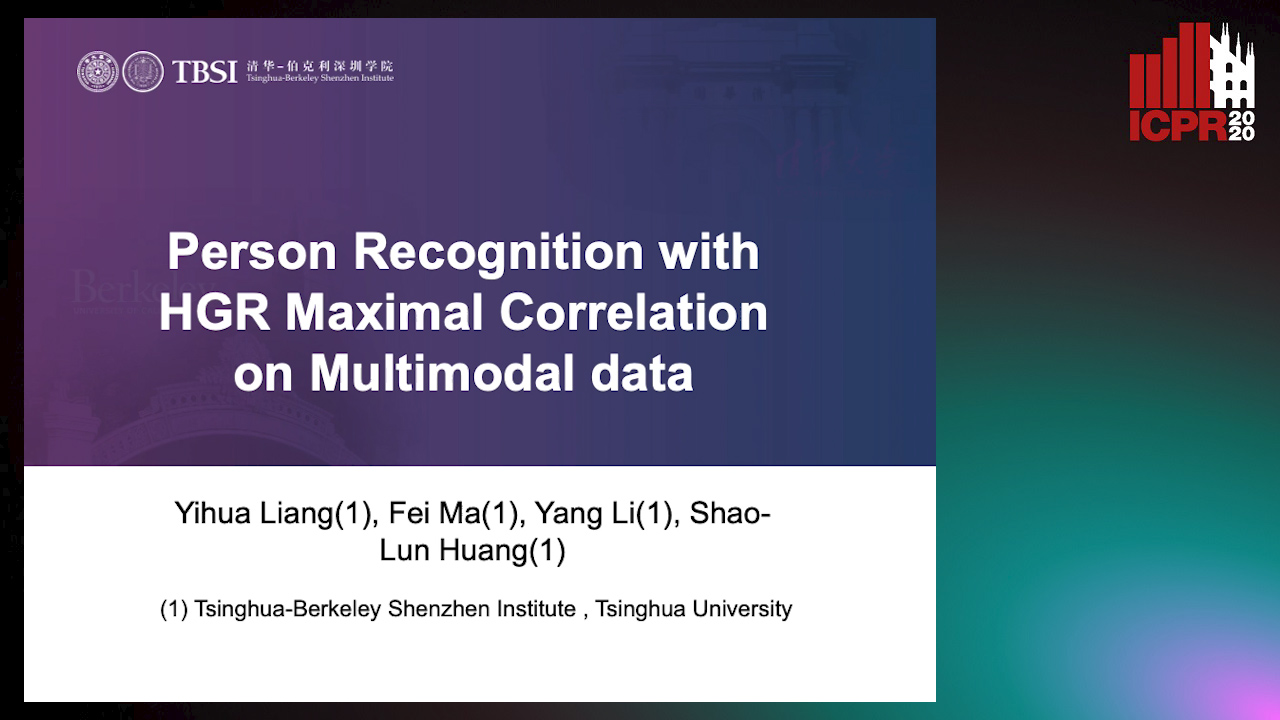
Auto-TLDR; A correlation-based multimodal person recognition framework that learns discriminative embeddings of persons by joint learning visual features and audio features
Abstract Slides Poster Similar
Multimodal person recognition is a common task in video analysis and public surveillance, where information from multiple modalities, such as images and audio extracted from videos, are used to jointly determine the identity of a person. Previous person recognition techniques either use only uni-modal data or only consider shared representations between different input modalities, while leaving the extraction of their relationship with identity information to downstream tasks. Furthermore, real-world data often contain noise, which makes recognition more challenging practical situations. In our work, we propose a novel correlation-based multimodal person recognition framework that is relatively simple but can efficaciously learn supervised information in multimodal data fusion and resist noise. Specifically, our framework learns a discriminative embeddings of persons by joint learning visual features and audio features while maximizing HGR maximal correlation among multimodal input and persons' identities. Experiments are done on a subset of Voxceleb2. Compared with state-of-the-art methods, the proposed method demonstrates an improvement of accuracy and robustness to noise.