Jaehyeong Cho
Paper download is intended for registered attendees only, and is
subjected to the IEEE Copyright Policy. Any other use is strongly forbidden.
Papers from this author
Mask-Based Style-Controlled Image Synthesis Using a Mask Style Encoder
Jaehyeong Cho, Wataru Shimoda, Keiji Yanai
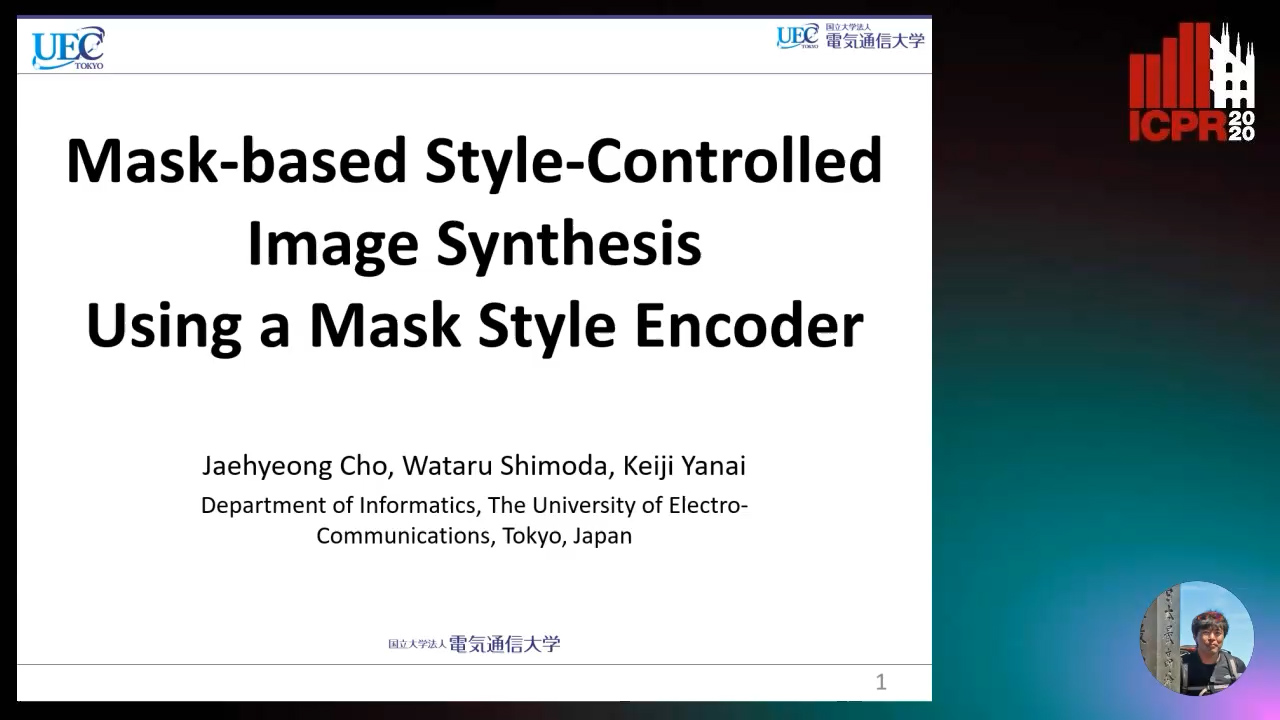
Auto-TLDR; Style-controlled Image Synthesis from Semantic Segmentation masks using GANs
Abstract Slides Poster Similar
In recent years, the advances in Generative Adversarial Networks (GANs) have shown impressive results for image generation and translation tasks. In particular, the image-to-image translation is a method of learning mapping from a source domain to a target domain and synthesizing an image. Image-to-image translation can be applied to a variety of tasks, making it possible to quickly and easily synthesize realistic images from semantic segmentation masks. However, in the existing image-to-image translation method, there is a limitation on controlling the style of the translated image, and it is not easy to synthesize an image by controlling the style of each mask element in detail. Therefore, we propose an image synthesis method that controls the style of each element by improving the existing image-to-image translation method. In the proposed method, we implement a style encoder that extracts style features for each mask element. The extracted style features are concatenated to the semantic mask in the normalization layer, and used the style-controlled image synthesis of each mask element. In experiments, we train style-controlled images synthesis using the datasets consisting of semantic segmentation masks and real images. The results show that the proposed method has excellent performance for style-controlled images synthesis for each element.