Zhenmao Li
Paper download is intended for registered attendees only, and is
subjected to the IEEE Copyright Policy. Any other use is strongly forbidden.
Papers from this author
Face Image Quality Assessment for Model and Human Perception
Ken Chen, Yichao Wu, Zhenmao Li, Yudong Wu, Ding Liang
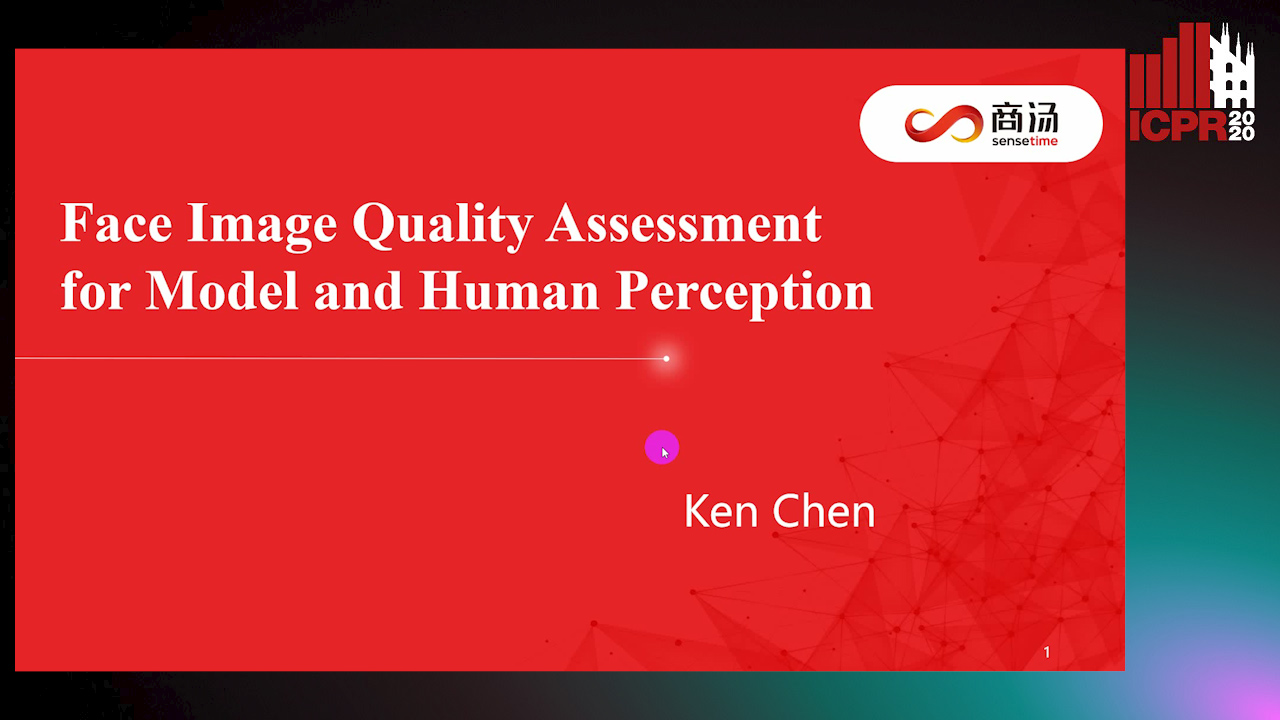
Auto-TLDR; A labour-saving method for FIQA training with contradictory data from multiple sources
Abstract Slides Poster Similar
Practical face image quality assessment (FIQA) models are trained under the supervision of labeled data, which requires more or less human labor. The human labeled quality scores are consistent with perceptual intuition but laborious. On the other hand, models can be trained with data generated automatically by the recognition models with artificially selected references. However, the recognition scores are sometimes inaccurate, which may give wrong quality scores during FIQA training. In this paper, we propose a labour-saving method for quality scores generation. For the first time, we conduct systematic investigations to show that there exist severe contradictions between different types of target quality, namely distribution gap (DG). To bridge the gap, we propose a novel framework for training FIQA models by combining the merits of data from different sources. In order to make the target score from multiple sources compatible, we design a method called quality distribution alignment (QDA). Meanwhile, to correct the wrong target by recognition models, contradictory samples selection (CSS) is adopted to select samples from the human labeled dataset adaptively. Extensive experiments and analysis on public benchmarks including MegaFace has demonstrated the superiority of our in terms of effectiveness and efficiency.