Jiaying Lin
Paper download is intended for registered attendees only, and is
subjected to the IEEE Copyright Policy. Any other use is strongly forbidden.
Papers from this author
Coherence and Identity Learning for Arbitrary-Length Face Video Generation
Shuquan Ye, Chu Han, Jiaying Lin, Guoqiang Han, Shengfeng He
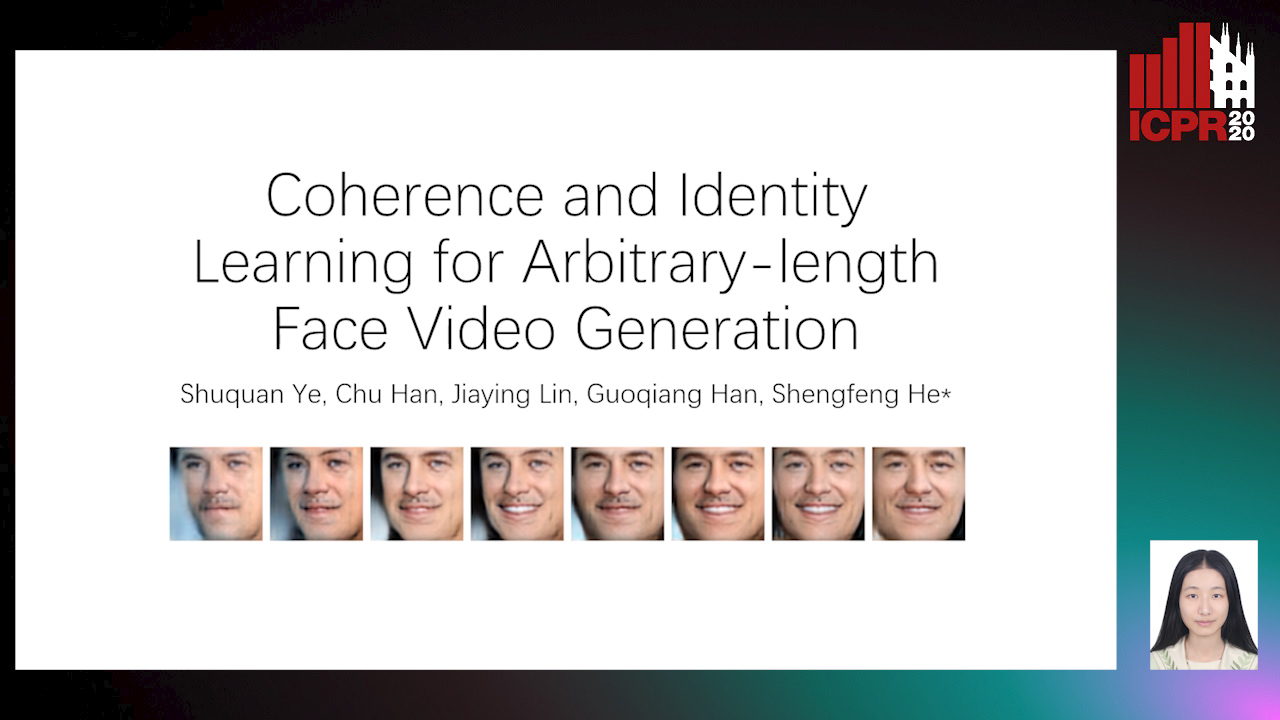
Auto-TLDR; Face Video Synthesis Using Identity-Aware GAN and Face Coherence Network
Abstract Slides Poster Similar
Face synthesis is an interesting yet challenging task in computer vision. It is even much harder to generate a portrait video than a single image. In this paper, we propose a novel video generation framework for synthesizing arbitrary-length face videos without any face exemplar or landmark. To overcome the synthesis ambiguity of face video, we propose a divide-and-conquer strategy to separately address the video face synthesis problem from two aspects, face identity synthesis and rearrangement. To this end, we design a cascaded network which contains three components, Identity-aware GAN (IA-GAN), Face Coherence Network, and Interpolation Network. IA-GAN is proposed to synthesize photorealistic faces with the same identity from a set of noises. Face Coherence Network is designed to re-arrange the faces generated by IA-GAN while keeping the inter-frame coherence. Interpolation Network is introduced to eliminate the discontinuity between two adjacent frames and improve the smoothness of the face video. Experimental results demonstrate that our proposed network is able to generate face video with high visual quality while preserving the identity. Statistics show that our method outperforms state-of-the-art unconditional face video generative models in multiple challenging datasets.