Teo Spadotto
Paper download is intended for registered attendees only, and is
subjected to the IEEE Copyright Policy. Any other use is strongly forbidden.
Papers from this author
Unsupervised Domain Adaptation with Multiple Domain Discriminators and Adaptive Self-Training
Teo Spadotto, Marco Toldo, Umberto Michieli, Pietro Zanuttigh
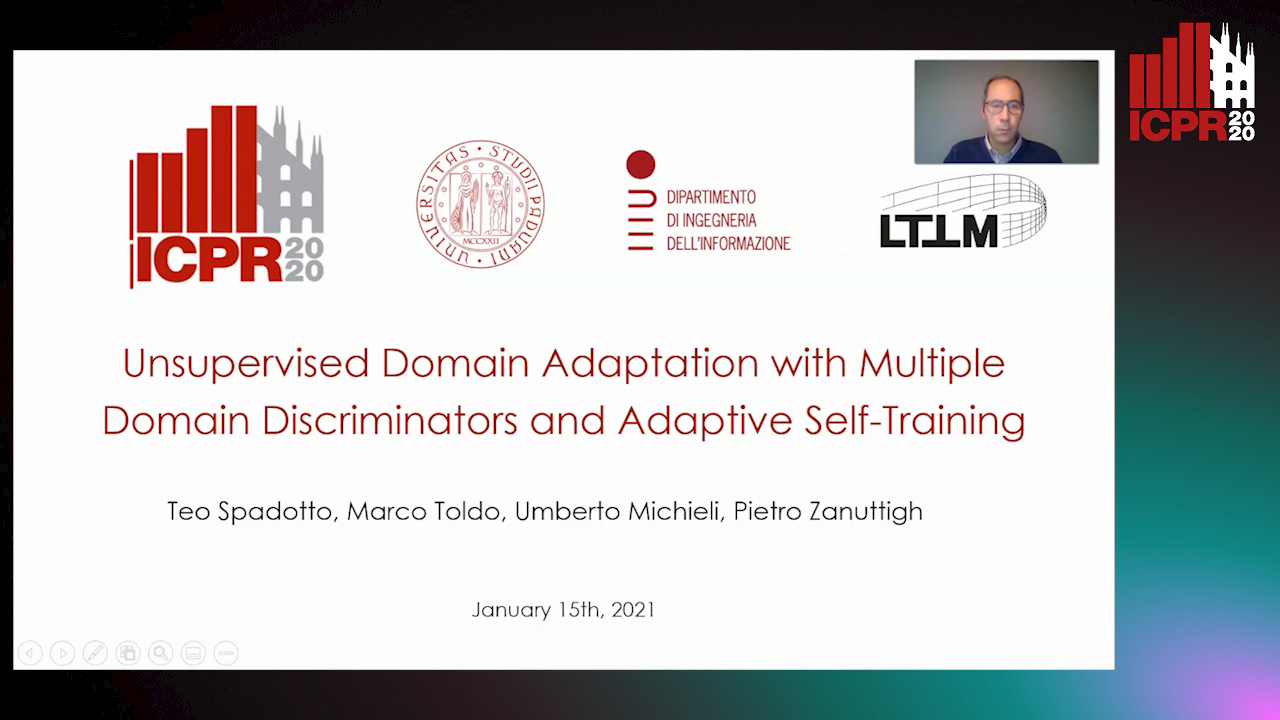
Auto-TLDR; Unsupervised Domain Adaptation for Semantic Segmentation of Urban Scenes
Abstract Slides Poster Similar
Unsupervised Domain Adaptation (UDA) aims at improving the generalization capability of a model trained on a source domain to perform well on a target domain for which no labeled data is available. In this paper, we consider the semantic segmentation of urban scenes and we propose an approach to adapt a deep neural network trained on synthetic data to real scenes addressing the domain shift between the two different data distributions. We introduce a novel UDA framework where a standard supervised loss on labeled synthetic data is supported by an adversarial module and a self-training strategy aiming at aligning the two domain distributions. The adversarial module is driven by a couple of fully convolutional discriminators dealing with different domains: the first discriminates between ground truth and generated maps, while the second between segmentation maps coming from synthetic or real world data. The self-training module exploits the confidence estimated by the discriminators on unlabeled data to select the regions used to reinforce the learning process. Furthermore, the confidence is thresholded with an adaptive mechanism based on the per-class overall confidence. Experimental results prove the effectiveness of the proposed strategy in adapting a segmentation network trained on synthetic datasets like GTA5 and SYNTHIA, to real world datasets like Cityscapes and Mapillary.