Austin Lawson
Paper download is intended for registered attendees only, and is
subjected to the IEEE Copyright Policy. Any other use is strongly forbidden.
Papers from this author
A Hybrid Metric Based on Persistent Homology and Its Application to Signal Classification
Austin Lawson, Yu-Min Chung, William Cruse
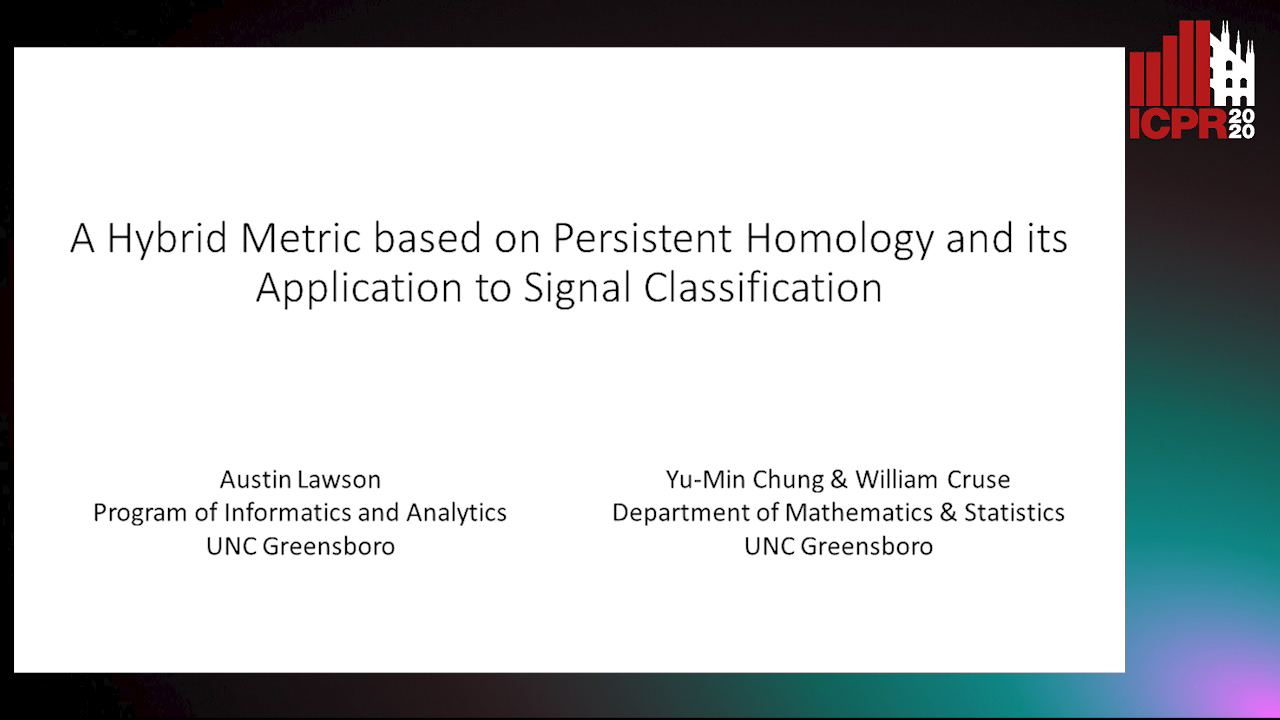
Auto-TLDR; Topological Data Analysis with Persistence Curves
Topological Data Analysis (TDA) is a rising field in machine learning. TDA considers the shape of data set. Persistence diagrams, one of main tools in TDA, store topological information about the data. Persistence curves, a recently developed framework, provides a canonical and flexible way to encode the information presented in persistence diagrams into vectors. Based on persistence curves, we (1) provide new sets of features for time series, (2) prove that these features are robust to noise, (3) propose a hybrid metric that takes both geometric and topological information of the time series into account. Finally, we apply these metrics to the UCR Time Series Classification Archive. These empirical results show that our metrics perform better than the relevant benchmark in most cases.