Seiichi Mita
Paper download is intended for registered attendees only, and is
subjected to the IEEE Copyright Policy. Any other use is strongly forbidden.
Papers from this author
Enhancing Depth Quality of Stereo Vision Using Deep Learning-Based Prior Information of the Driving Environment
Weifu Li, Vijay John, Seiichi Mita
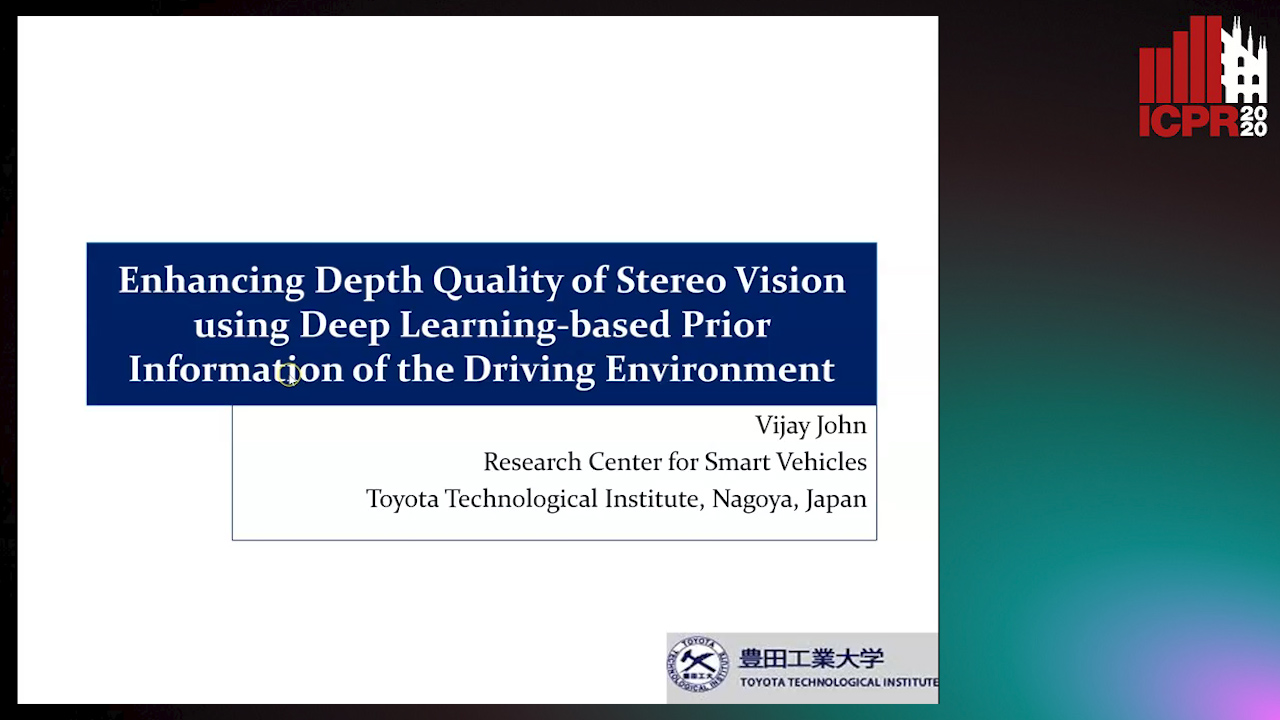
Auto-TLDR; A Novel Post-processing Mathematical Framework for Stereo Vision
Abstract Slides Poster Similar
Generation of high density depth values of the driving environment is indispensable for autonomous driving. Stereo vision is one of the practical and effective methods to generate these depth values. However, the accuracy of the stereo vision is limited by texture-less regions, such as sky and road areas, and repeated patterns in the image. To overcome these problems, we propose to enhance the stereo generated depth by incorporating prior information of the driving environment. Prior information, generated by deep learning-based U-Net model, is utilized in a novel post-processing mathematical framework to refine the stereo generated depth. The proposed mathematical framework is formulated as an optimization problem, which refines the errors due to texture-less regions and repeated patterns. Owing to its mathematical formulation, the post-processing framework is not a black-box and is explainable, and can be readily utilized for depth maps generated by any stereo vision algorithm. The proposed framework is qualitatively validated on the acquired dataset and KITTI dataset. The results obtained show that the proposed framework improves the stereo depth generation accuracy