Zhaohui Wang
Paper download is intended for registered attendees only, and is
subjected to the IEEE Copyright Policy. Any other use is strongly forbidden.
Papers from this author
ACCLVOS: Atrous Convolution with Spatial-Temporal ConvLSTM for Video Object Segmentation
Muzhou Xu, Shan Zong, Chunping Liu, Shengrong Gong, Zhaohui Wang, Yu Xia
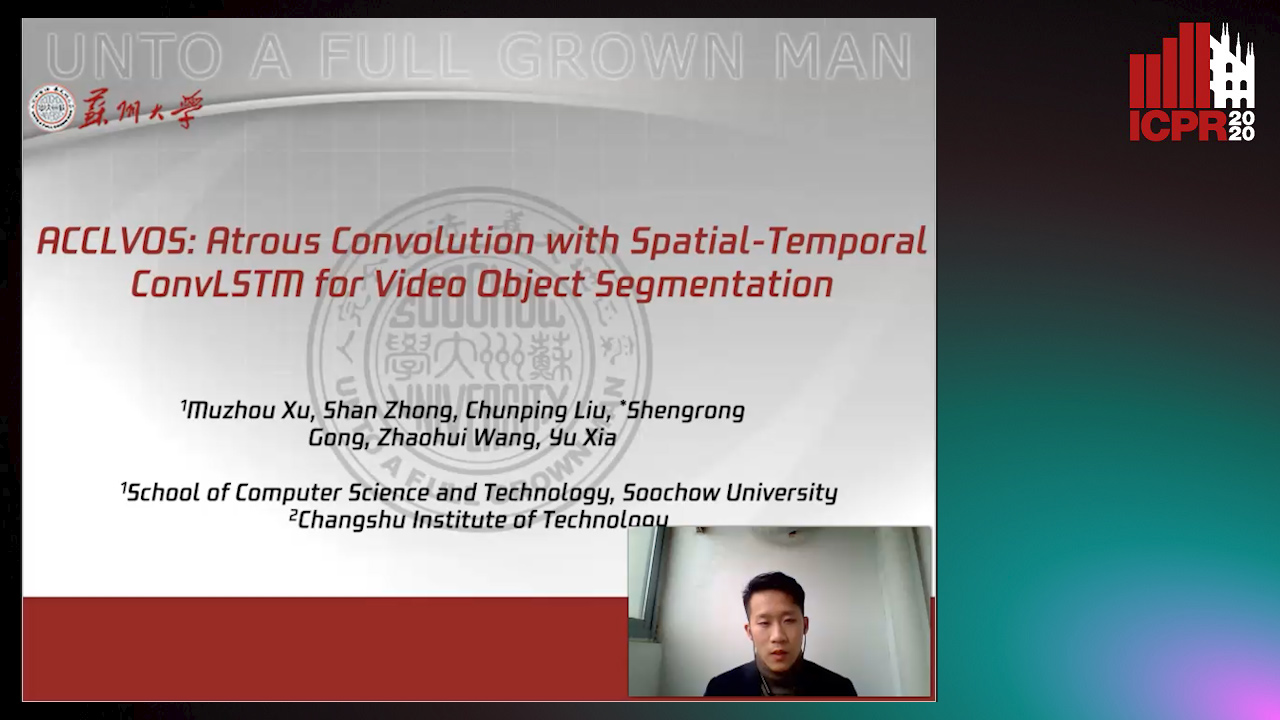
Auto-TLDR; Semi-supervised Video Object Segmentation using U-shape Convolution and ConvLSTM
Abstract Slides Poster Similar
Semi-supervised video object segmentation aims at segmenting the target of interest throughout a video sequence when only the annotated mask of the first frame is given. A feasible method for segmentation is to capture the spatial-temporal coherence between frames. However, it may suffer from mask drift when the spatial-temporal coherence is unreliable. To relieve this problem, we propose an encoder-decoder-recurrent model for semi-supervised video object segmentation. The model adopts a U-shape architecture that combines atrous convolution and ConvLSTM to establish the coherence in both the spatial and temporal domains. Furthermore, the weight ratio for each block is also reconstructed to make the model more suitable for the VOS task. We evaluate our method on two benchmarks, DAVIS-2017 and Youtube-VOS, where state-of-the-art segmentation accuracy with a real-time inference speed of 21.3 frames per second on a Tesla P100 is obtained.