Pierre-Etienne Martin
Paper download is intended for registered attendees only, and is
subjected to the IEEE Copyright Policy. Any other use is strongly forbidden.
Papers from this author
3D Attention Mechanism for Fine-Grained Classification of Table Tennis Strokes Using a Twin Spatio-Temporal Convolutional Neural Networks
Pierre-Etienne Martin, Jenny Benois-Pineau, Renaud Péteri, Julien Morlier
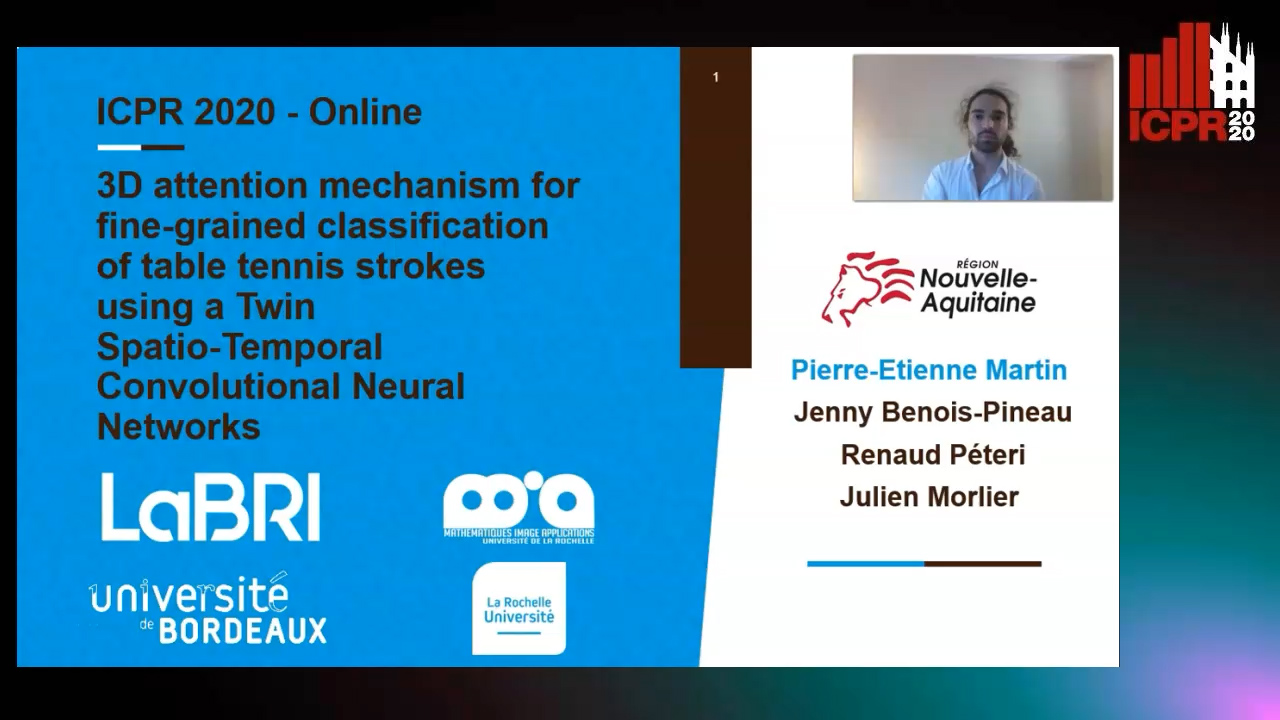
Auto-TLDR; Attentional Blocks for Action Recognition in Table Tennis Strokes
Abstract Slides Poster Similar
The paper addresses the problem of recognition of actions in video with low inter-class variability such as Table Tennis strokes. Two stream, "twin" convolutional neural networks are used with 3D convolutions both on RGB data and optical flow. Actions are recognized by classification of temporal windows. We introduce 3D attention modules and examine their impact on classification efficiency. In the context of the study of sportsmen performances, a corpus of the particular actions of table tennis strokes is considered. The use of attention blocks in the network speeds up the training step and improves the classification scores up to 5% with our twin model. We visualize the impact on the obtained features and notice correlation between attention and player movements and position. Score comparison of state-of-the-art action classification method and proposed approach with attentional blocks is performed on the corpus. Proposed model with attention blocks outperforms previous model without them and our baseline.