Mathias Seuret
Paper download is intended for registered attendees only, and is
subjected to the IEEE Copyright Policy. Any other use is strongly forbidden.
Papers from this author
Trainable Spectrally Initializable Matrix Transformations in Convolutional Neural Networks
Michele Alberti, Angela Botros, Schuetz Narayan, Rolf Ingold, Marcus Liwicki, Mathias Seuret
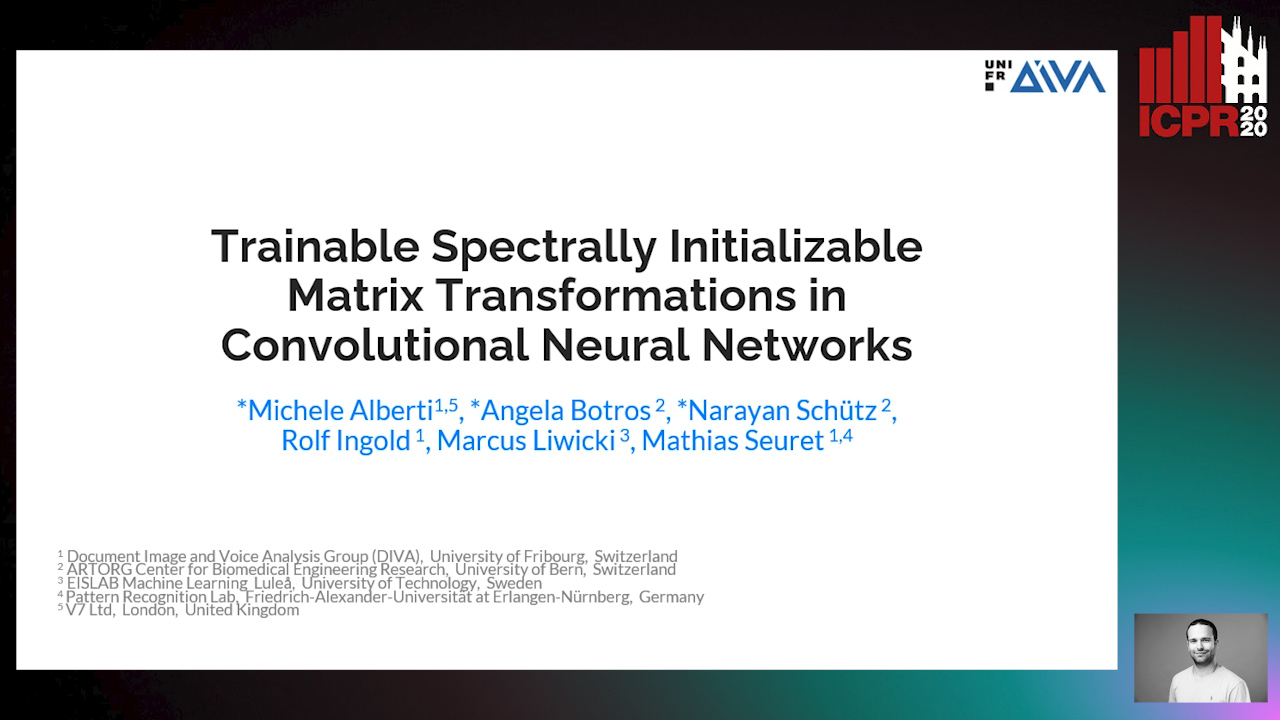
Auto-TLDR; Trainable and Spectrally Initializable Matrix Transformations for Neural Networks
Abstract Slides Poster Similar
In this work, we introduce a new architectural component to Neural Networks (NN), i.e., trainable and spectrally initializable matrix transformations on feature maps. While previous literature has already demonstrated the possibility of adding static spectral transformations as feature processors, our focus is on more general trainable transforms. We study the transforms in various architectural configurations on four datasets of different nature: from medical (ColorectalHist, HAM10000) and natural (Flowers) images to historical documents (CB55). With rigorous experiments that control for the number of parameters and randomness, we show that networks utilizing the introduced matrix transformations outperform vanilla neural networks. The observed accuracy increases appreciably across all datasets. In addition, we show that the benefit of spectral initialization leads to significantly faster convergence, as opposed to randomly initialized matrix transformations. The transformations are implemented as auto-differentiable PyTorch modules that can be incorporated into any neural network architecture. The entire code base is open-source.