Xiaolin Hu
Paper download is intended for registered attendees only, and is
subjected to the IEEE Copyright Policy. Any other use is strongly forbidden.
Papers from this author
Dynamic Multi-Path Neural Network
Yingcheng Su, Yichao Wu, Ken Chen, Ding Liang, Xiaolin Hu
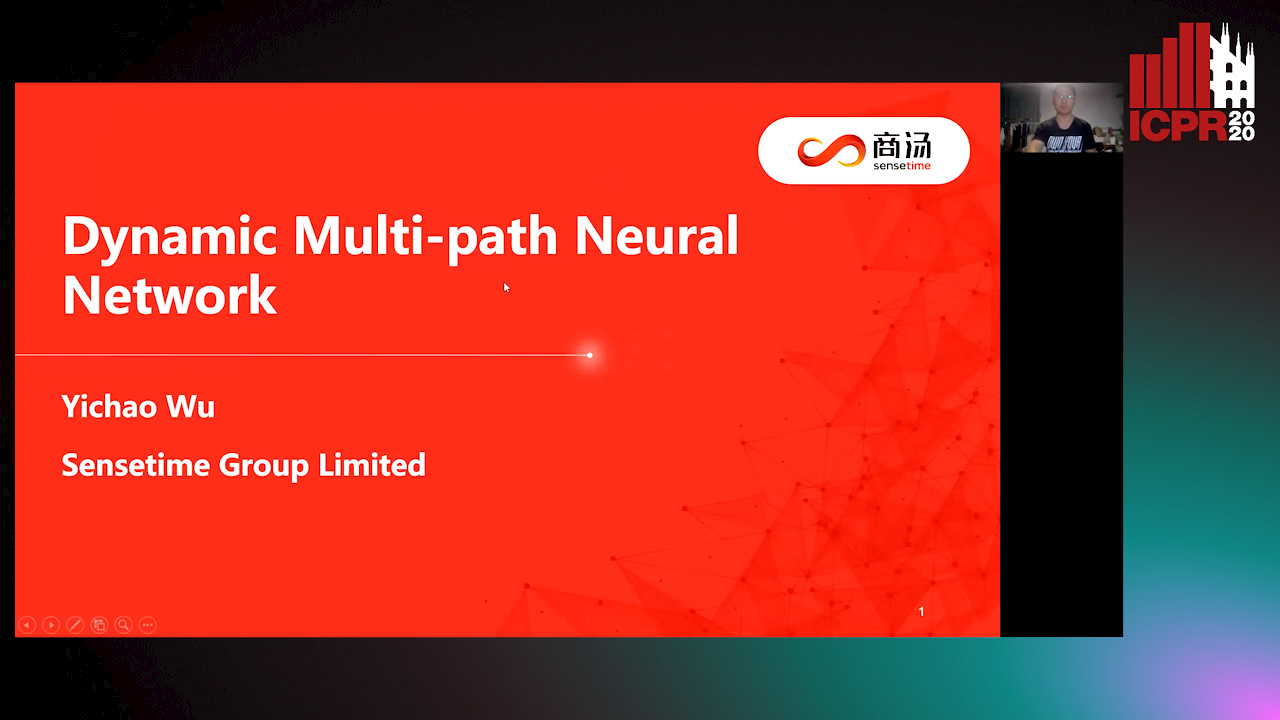
Auto-TLDR; Dynamic Multi-path Neural Network
Although deeper and larger neural networks have achieved better performance, due to overwhelming burden on computation, they cannot meet the demands of deployment on resource-limited devices. An effective strategy to address this problem is to make use of dynamic inference mechanism, which changes the inference path for different samples at runtime. Existing methods only reduce the depth by skipping an entire specific layer, which may lose important information in this layer. In this paper, we propose a novel method called Dynamic Multi-path Neural Network (DMNN), which provides more topology choices in terms of both width and depth on the fly. For better modelling the inference path selection, we further introduce previous state and object category information to guide the training process. Compared to previous dynamic inference techniques, the proposed method is more flexible and easier to incorporate into most modern network architectures. Experimental results on ImageNet and CIFAR-100 demonstrate the superiority of our method on both efficiency and classification accuracy.