Joost Van De Weijer
Paper download is intended for registered attendees only, and is
subjected to the IEEE Copyright Policy. Any other use is strongly forbidden.
Papers from this author
Learning to Rank for Active Learning: A Listwise Approach
Minghan Li, Xialei Liu, Joost Van De Weijer, Bogdan Raducanu
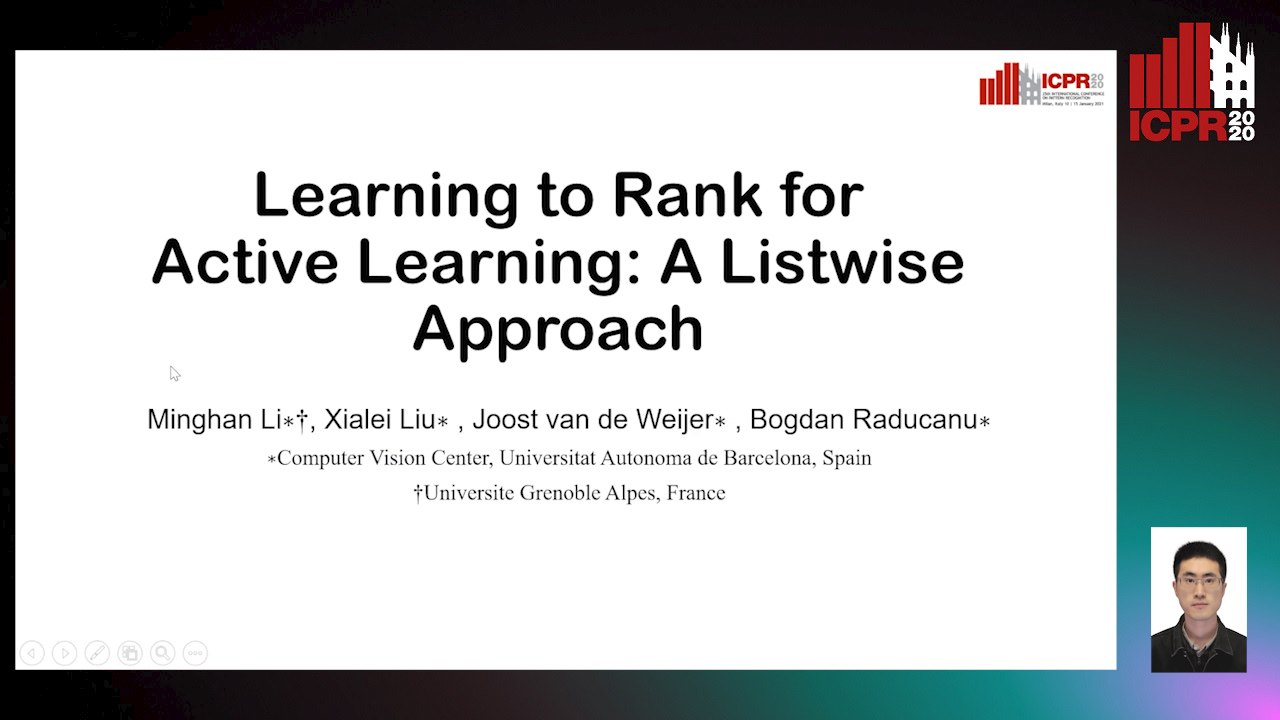
Auto-TLDR; Learning Loss for Active Learning
Active learning emerged as an alternative to alleviate the effort to label huge amount of data for data-hungry applications (such as image/video indexing and retrieval, autonomous driving, etc.). The goal of active learning is to automatically select a number of unlabeled samples for annotation (according to a budget), based on an acquisition function, which indicates how valuable a sample is for training the model. The learning loss method is a task-agnostic approach which attaches a module to learn to predict the target loss of unlabeled data, and select data with the highest loss for labeling. In this work, we follow this strategy but we define the acquisition function as a learning to rank problem and rethink the structure of the loss prediction module, using a simple but effective listwise approach. Experimental results on four datasets demonstrate that our method outperforms recent state-of-the-art active learning approaches for both image classification and regression tasks.