Peter Bellmann
Paper download is intended for registered attendees only, and is
subjected to the IEEE Copyright Policy. Any other use is strongly forbidden.
Papers from this author
Using Meta Labels for the Training of Weighting Models in a Sample-Specific Late Fusion Classification Architecture
Peter Bellmann, Patrick Thiam, Friedhelm Schwenker
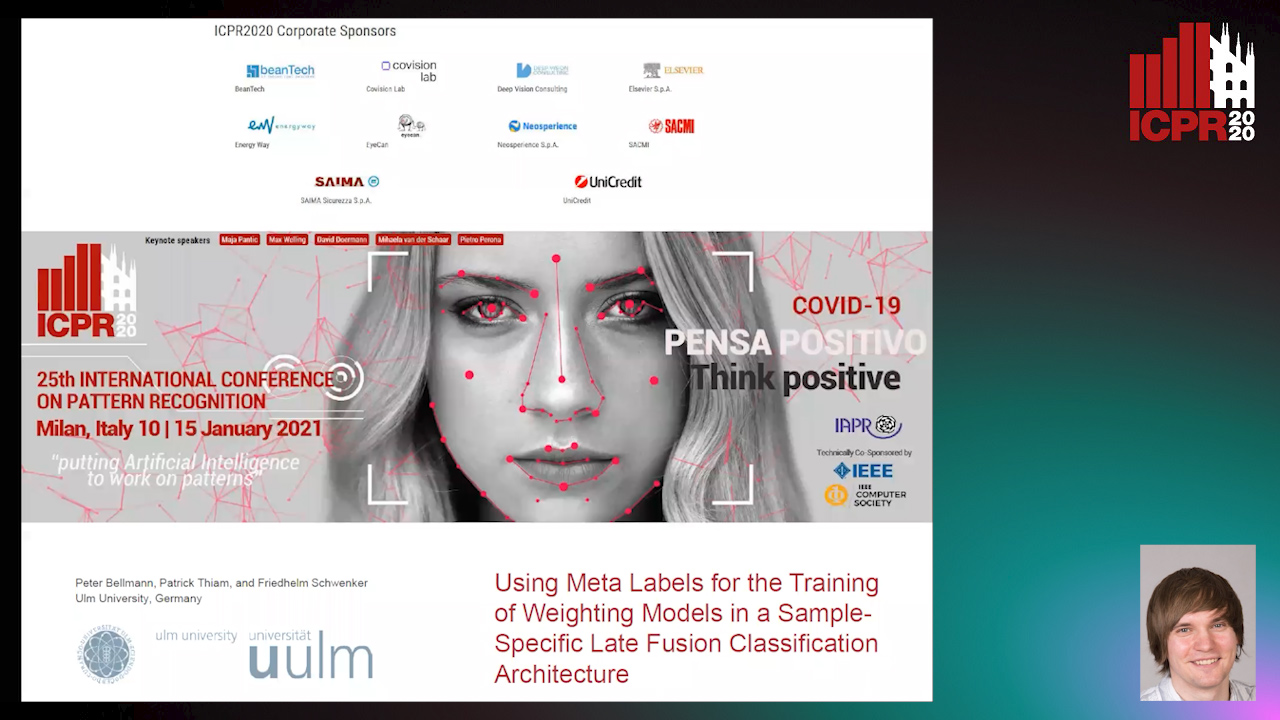
Auto-TLDR; A Late Fusion Architecture for Multiple Classifier Systems
Abstract Slides Poster Similar
The performance of multiple classifier systems can be significantly improved by the use of intelligent classifier combination approaches. In this study, we introduce a novel late fusion architecture, which can be interpreted as a combination of the well-known mixture of experts and stacked generalization methods. Our proposed method aggregates the outputs of classification models and corresponding sample-specific weighting models. A special feature of our proposed architecture is that each weighting model is trained on an individual set of meta labels. Using individual sets of meta labels allows each weighting model to separate regions, on which the predictions of the corresponding classification model can be associated to an estimated confidence value. We test our proposed architecture on a set of publicly available databases, including different benchmark data sets. The experimental evaluation shows the effectiveness and potential of our proposed method. Moreover, we discuss different approaches for further improvement of our proposed architecture.