Shengjie Zhao
Paper download is intended for registered attendees only, and is
subjected to the IEEE Copyright Policy. Any other use is strongly forbidden.
Papers from this author
Cross-Domain Semantic Segmentation of Urban Scenes Via Multi-Level Feature Alignment
Bin Zhang, Shengjie Zhao, Rongqing Zhang
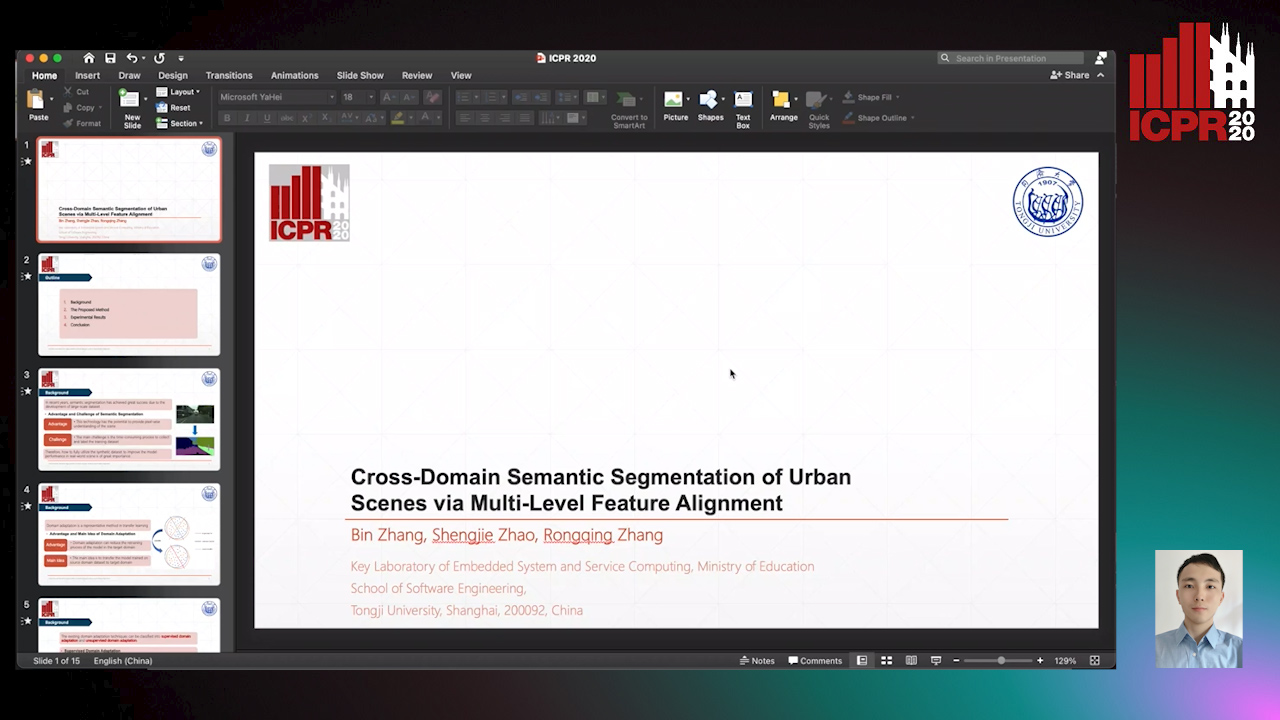
Auto-TLDR; Cross-Domain Semantic Segmentation Using Generative Adversarial Networks
Abstract Slides Poster Similar
Semantic segmentation is an essential task in plenty of real-life applications such as virtual reality, video analysis, autonomous driving, etc. Recent advancements in fundamental vision-based tasks ranging from image classification to semantic segmentation have demonstrated deep learning-based models' high capability in learning complicated representation on large datasets. Nevertheless, manually labeling semantic segmentation dataset with pixel-level annotation is extremely labor-intensive. To address this problem, we propose a novel multi-level feature alignment framework for cross-domain semantic segmentation of urban scenes by exploiting generative adversarial networks. In the proposed multi-level feature alignment method, we first translate images from one domain to another one. Then the discriminative feature representations extracted by the deep neural network are concatenated, followed by domain adversarial learning to make the intermediate feature distribution of the target domain images close to those in the source domain. With these domain adaptation techniques, models trained with images in the source domain where the labels are easy to acquire can be deployed to the target domain where the labels are scarce. Experimental evaluations on various mainstream benchmarks confirm the effectiveness as well as robustness of our approach.