Kunihito Kato
Paper download is intended for registered attendees only, and is
subjected to the IEEE Copyright Policy. Any other use is strongly forbidden.
Papers from this author
Disentangle, Assemble, and Synthesize: Unsupervised Learning to Disentangle Appearance and Location
Hiroaki Aizawa, Hirokatsu Kataoka, Yutaka Satoh, Kunihito Kato
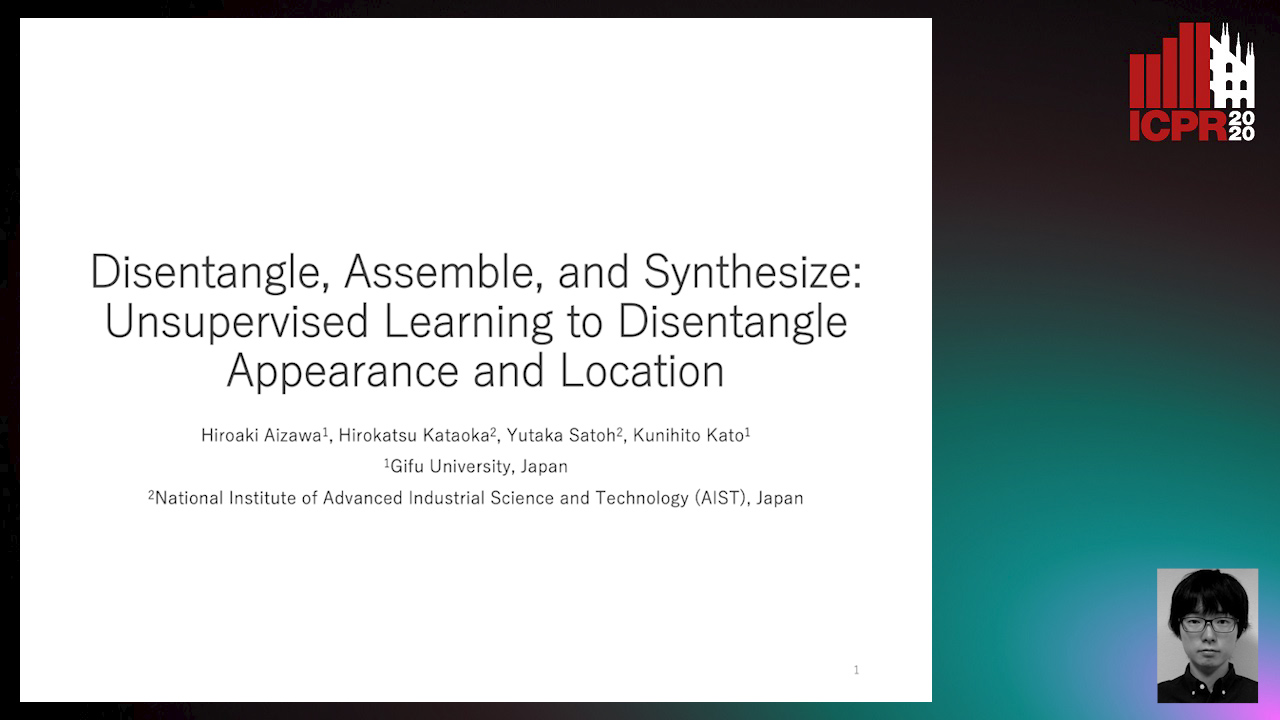
Auto-TLDR; Generative Adversarial Networks with Structural Constraint for controllability of latent space
Abstract Slides Poster Similar
The next step for the generative adversarial networks~(GAN) is to learn representations that allow us to control only a certain factor in the image explicitly. Since such a representation of the factor is independent of other factors, the controllability obtained from these representations leads to interpretability by identifying the variation of the synthesized image and the transferability for downstream tasks by inference. However, since it is difficult to identify and strictly define latent factors, the annotation is laborious. Moreover, learning such representations by a GAN is challenging due to the complex generation process. Therefore, we resolve this limitation using a novel generative model that can disentangle latent space into the appearance, the x-axis, and the y-axis of the object, and reassemble these components in an unsupervised manner. Specifically, based on the concept of packing the appearance and location in each position of the feature map, we introduce a novel structural constraint technique that prevents these representations from interacting with each other. The proposed structural constraint promotes the disentanglement of these factors. In experiments, we found that the proposed method is simple but effective for controllability and allows us to control the appearance and location via latent space without supervision, as compared with the conditional GAN.