Zhen Yang
Paper download is intended for registered attendees only, and is
subjected to the IEEE Copyright Policy. Any other use is strongly forbidden.
Papers from this author
Manual-Label Free 3D Detection Via an Open-Source Simulator
Zhen Yang, Chi Zhang, Zhaoxiang Zhang, Huiming Guo
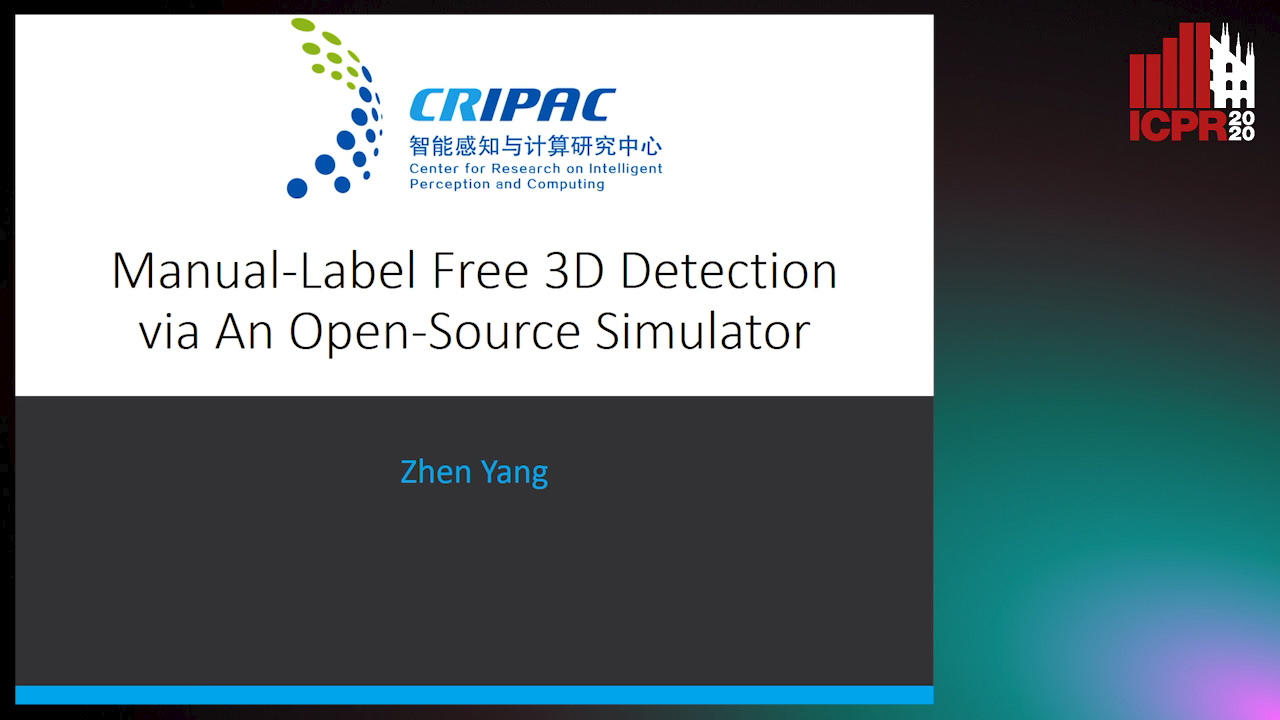
Auto-TLDR; DA-VoxelNet: A Novel Domain Adaptive VoxelNet for LIDAR-based 3D Object Detection
Abstract Slides Poster Similar
LiDAR based 3D object detectors typically need a large amount of detailed-labeled point cloud data for training, but these detailed labels are commonly expensive to acquire. In this paper, we propose a manual-label free 3D detection algorithm that leverages the CARLA simulator to generate a large amount of self-labeled training samples and introduces a novel Domain Adaptive VoxelNet (DA-VoxelNet) that can cross the distribution gap from the synthetic data to the real scenario. The self-labeled training samples are generated by a set of high quality 3D models embedded in a CARLA simulator and a proposed LiDAR-guided sampling algorithm. Then a DA-VoxelNet that integrates both a sample-level DA module and an anchor-level DA module is proposed to enable the detector trained by the synthetic data to adapt to real scenario. Experimental results show that the proposed unsupervised DA 3D detector on KITTI evaluation set can achieve 76.66% and 56.64% mAP on BEV mode and 3D mode respectively. The results reveal a promising perspective of training a LIDAR-based 3D detector without any hand-tagged label.