Jason Allen
Paper download is intended for registered attendees only, and is
subjected to the IEEE Copyright Policy. Any other use is strongly forbidden.
Papers from this author
SAGE: Sequential Attribute Generator for Analyzing Glioblastomas Using Limited Dataset
Padmaja Jonnalagedda, Brent Weinberg, Jason Allen, Taejin Min, Shiv Bhanu, Bir Bhanu
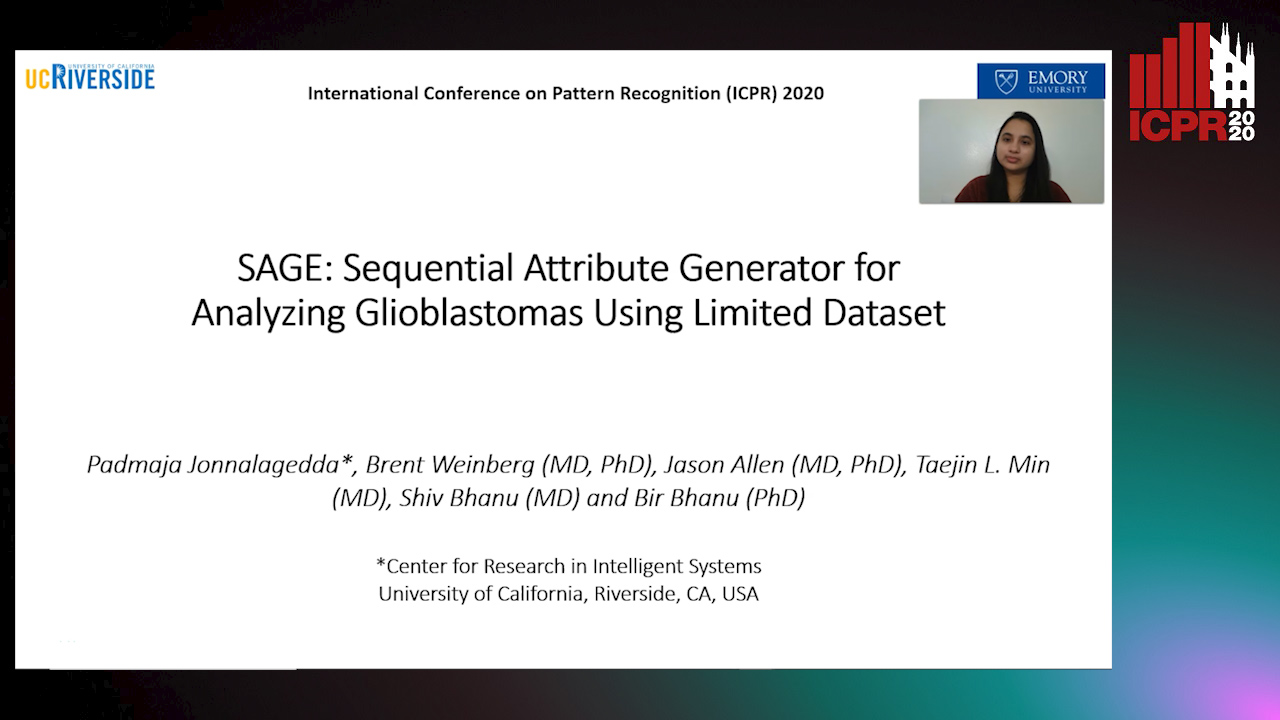
Auto-TLDR; SAGE: Generative Adversarial Networks for Imaging Biomarker Detection and Prediction
Abstract Slides Poster Similar
While deep learning approaches have shown remarkable performance in many imaging tasks, most of these methods rely on availability of large quantities of data. Medical image data, however, is scarce and fragmented. Generative Adversarial Networks (GANs) have recently been very effective in handling such datasets by generating more data. If the datasets are very small, however, GANs cannot learn the data distribution properly, resulting in less diverse or low-quality results. One such limited dataset is that for the concurrent gain of 19/20 chromosomes (19/20 co-gain), a mutation with positive prognostic value in Glioblastomas (GBM). In this paper, we detect imaging biomarkers for the mutation to streamline the extensive and invasive prognosis pipeline. Since this mutation is relatively rare, i.e. small dataset, we propose a novel generative framework – the Sequential Attribute GEnerator (SAGE), that generates detailed tumor imaging features while learning from a limited dataset. Experiments show that not only does SAGE generate high quality tumors when compared to standard Deep Convolutional GAN (DC-GAN) and Wasserstein GAN with Gradient Penalty (WGAN-GP), it also captures the imaging biomarkers accurately.