Jie Wen
Paper download is intended for registered attendees only, and is
subjected to the IEEE Copyright Policy. Any other use is strongly forbidden.
Papers from this author
Joint Learning Multiple Curvature Descriptor for 3D Palmprint Recognition
Lunke Fei, Bob Zhang, Jie Wen, Chunwei Tian, Peng Liu, Shuping Zhao
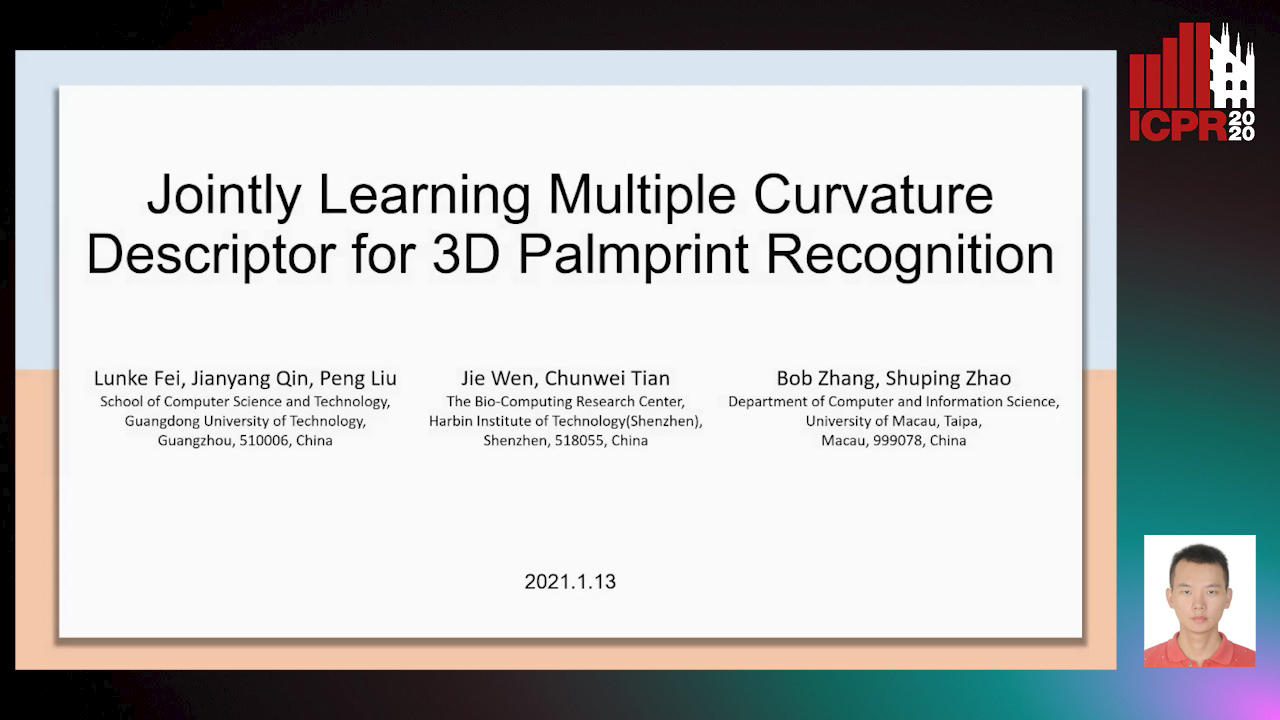
Auto-TLDR; Joint Feature Learning for 3D palmprint recognition using curvature data vectors
Abstract Slides Poster Similar
3D palmprint-based biometric recognition has drawn growing research attention due to its several merits over 2D counterpart such as robust structural measurement of a palm surface and high anti-counterfeiting capability. However, most existing 3D palmprint descriptors are hand-crafted that usually extract stationary features from 3D palmprint images. In this paper, we propose a feature learning method to jointly learn compact curvature feature descriptor for 3D palmprint recognition. We first form multiple curvature data vectors to completely sample the intrinsic curvature information of 3D palmprint images. Then, we jointly learn a feature projection function that project curvature data vectors into binary feature codes, which have the maximum inter-class variances and minimum intra-class distance so that they are discriminative. Moreover, we learn the collaborative binary representation of the multiple curvature feature codes by minimizing the information loss between the final representation and the multiple curvature features, so that the proposed method is more compact in feature representation and efficient in matching. Experimental results on the baseline 3D palmprint database demonstrate the superiority of the proposed method in terms of recognition performance in comparison with state-of-the-art 3D palmprint descriptors.