Suekyeong Nam
Paper download is intended for registered attendees only, and is
subjected to the IEEE Copyright Policy. Any other use is strongly forbidden.
Papers from this author
JT-MGCN: Joint-Temporal Motion Graph Convolutional Network for Skeleton-Based Action Recognition
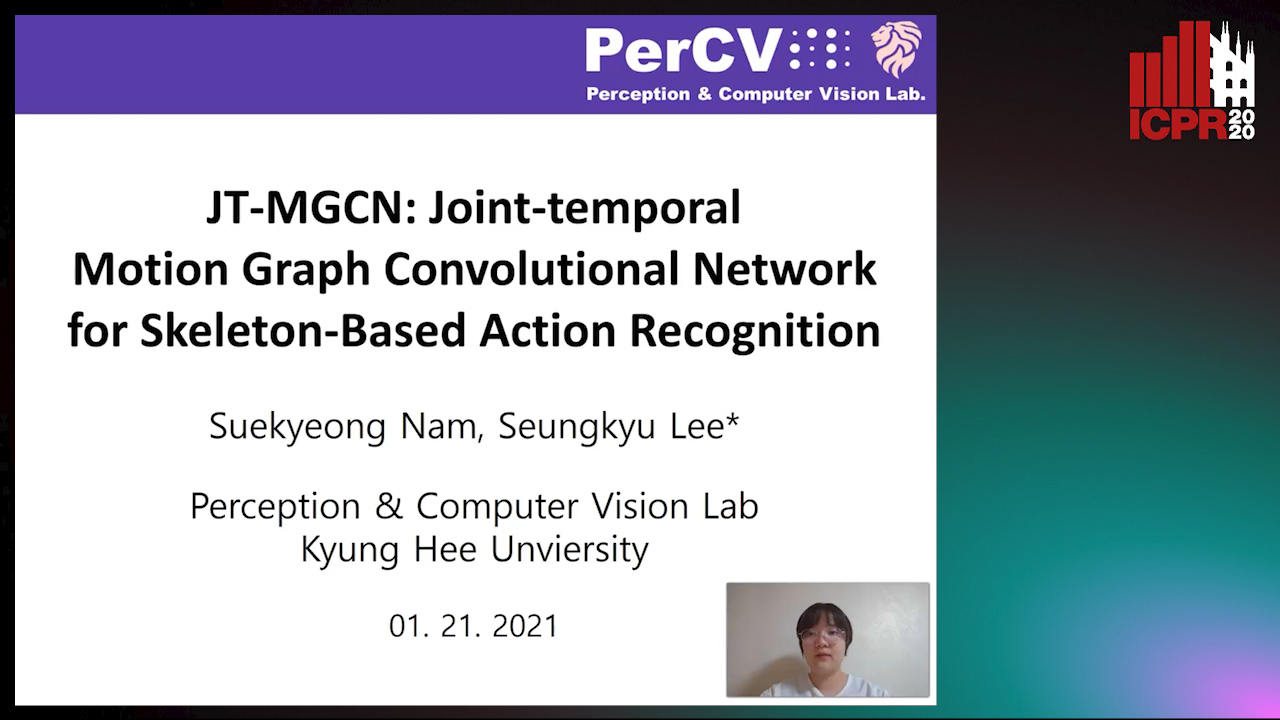
Auto-TLDR; Joint-temporal Motion Graph Convolutional Networks for Action Recognition
Recently, action recognition methods using graph convolutional networks (GCN) have shown remarkable performance thanks to its concise but effective representation of human body motion. Prior methods construct human body motion graph building edges between neighbor or distant body joints. On the other hand, human action contains lots of temporal variations showing strong temporal correlations between joint motions. Thus the characterization of an action requires a comprehensive analysis of joint motion correlations on spatial and temporal domains. In this paper, we propose Joint-temporal Motion Graph Convolutional Networks (JT-MGCN) in which joint-temporal edges learn the correlations between different joints at different time. Experimental evaluation on large public data sets such as NTU rgb+d data set and kinetics-skeleton data set show outstanding action recognition performance.