Michael Greenspan
Paper download is intended for registered attendees only, and is
subjected to the IEEE Copyright Policy. Any other use is strongly forbidden.
Papers from this author
Two-Level Attention-Based Fusion Learning for RGB-D Face Recognition
Hardik Uppal, Alireza Sepas-Moghaddam, Michael Greenspan, Ali Etemad
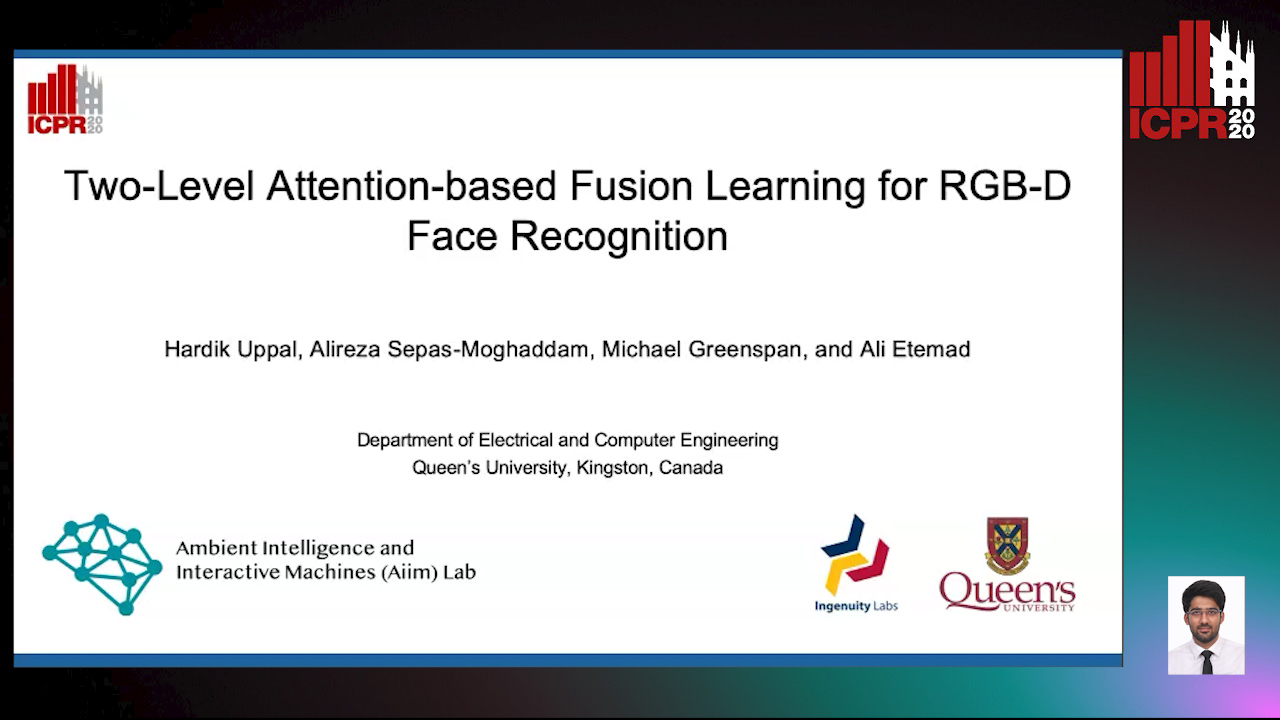
Auto-TLDR; Fused RGB-D Facial Recognition using Attention-Aware Feature Fusion
Abstract Slides Poster Similar
With recent advances in RGB-D sensing technologies as well as improvements in machine learning and fusion techniques, RGB-D facial recognition has become an active area of research. A novel attention aware method is proposed to fuse two image modalities, RGB and depth, for enhanced RGB-D facial recognition. The proposed method first extracts features from both modalities using a convolutional feature extractor. These features are then fused using a two layer attention mechanism. The first layer focuses on the fused feature maps generated by the feature extractor, exploiting the relationship between feature maps using LSTM recurrent learning. The second layer focuses on the spatial features of those maps using convolution. The training database is preprocessed and augmented through a set of geometric transformations, and the learning process is further aided using transfer learning from a pure 2D RGB image training process. Comparative evaluations demonstrate that the proposed method outperforms other state-of-the-art approaches, including both traditional and deep neural network-based methods, on the challenging CurtinFaces and IIIT-D RGB-D benchmark databases, achieving classification accuracies over 98.2% and 99.3% respectively. The proposed attention mechanism is also compared with other attention mechanisms, demonstrating more accurate results.