Ignacio Serna
Paper download is intended for registered attendees only, and is
subjected to the IEEE Copyright Policy. Any other use is strongly forbidden.
Papers from this author
InsideBias: Measuring Bias in Deep Networks and Application to Face Gender Biometrics
Ignacio Serna, Alejandro Peña Almansa, Aythami Morales, Julian Fierrez
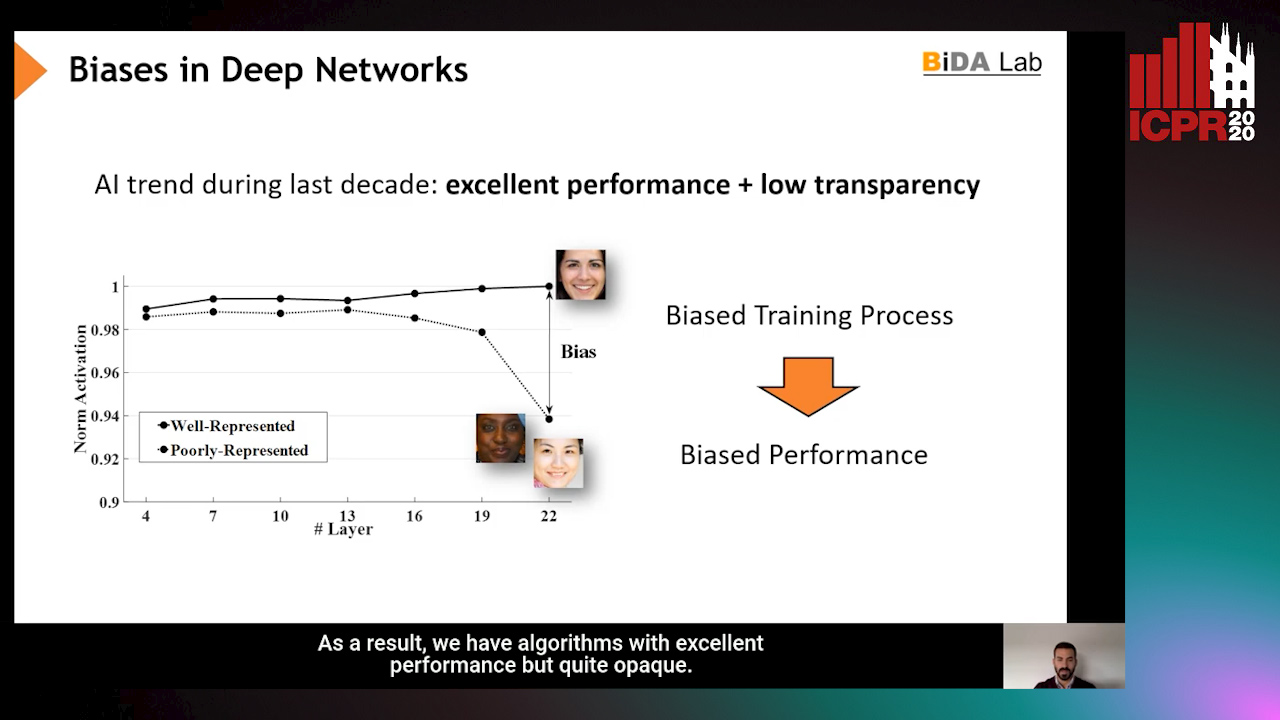
Auto-TLDR; InsideBias: Detecting Bias in Deep Neural Networks from Face Images
Abstract Slides Poster Similar
This work explores the biases in learning processes based on deep neural network architectures. We analyze how bias affects deep learning processes through a toy example using the MNIST database and a case study in gender detection from face images. We employ two gender detection models based on popular deep neural networks. We present a comprehensive analysis of bias effects when using an unbalanced training dataset on the features learned by the models. We show how bias impacts in the activations of gender detection models based on face images. We finally propose InsideBias, a novel method to detect biased models. InsideBias is based on how the models represent the information instead of how they perform, which is the normal practice in other existing methods for bias detection. Our strategy with InsideBias allows to detect biased models with very few samples (only 15 images in our case study). Our experiments include 72K face images from 24K identities and 3 ethnic groups.