Xudong Chen
Paper download is intended for registered attendees only, and is
subjected to the IEEE Copyright Policy. Any other use is strongly forbidden.
Papers from this author
A Cross Domain Multi-Modal Dataset for Robust Face Anti-Spoofing
Qiaobin Ji, Shugong Xu, Xudong Chen, Shan Cao, Shunqing Zhang
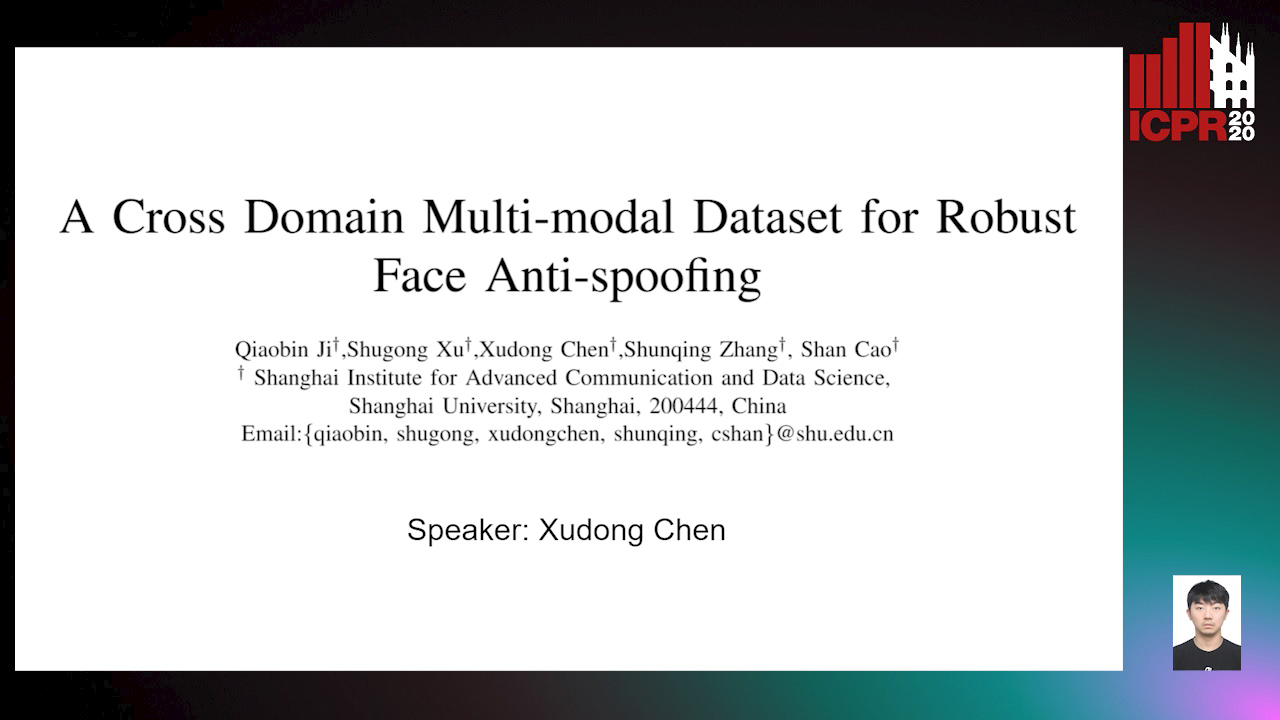
Auto-TLDR; Cross domain multi-modal FAS dataset GREAT-FASD and several evaluation protocols for academic community
Abstract Slides Poster Similar
Face Anti-spoofing (FAS) is a challenging problem due to the complex serving scenario and diverse face presentation attack patterns. Using single modal images which are usually captured with RGB cameras is not able to deal with the former because of serious overfitting problems. The existing multi-modal FAS datasets rarely pay attention to the cross domain problems, trainingFASsystemonthesedataleadstoinconsistenciesandlow generalization capabilities in deployment since imaging principles(structured light, TOF, etc.) and pre-processing methods vary between devices. We explore the subtle fine-grained differences betweeen multi-modal cameras and proposed a cross domain multi-modal FAS dataset GREAT-FASD and several evaluation protocols for academic community. Furthermore, we incorporate the multiplicative attention and center loss to enhance the representative power of CNN via seeking out complementary information as a powerful baseline. In addition, extensive experiments have been conducted on the proposed dataset to analyze the robustness to distinguish spoof faces and bona-fide faces. Experimental results show the effectiveness of proposed method and achieve the state-of-the-art competitive results. Finally, we visualize our future distribution in hidden space and observe that the proposed method is able to lead the network to generate a large margin for face anti-spoofing task