Chiara Pero
Paper download is intended for registered attendees only, and is
subjected to the IEEE Copyright Policy. Any other use is strongly forbidden.
Papers from this author
HP2IFS: Head Pose Estimation Exploiting Partitioned Iterated Function Systems
Carmen Bisogni, Michele Nappi, Chiara Pero, Stefano Ricciardi
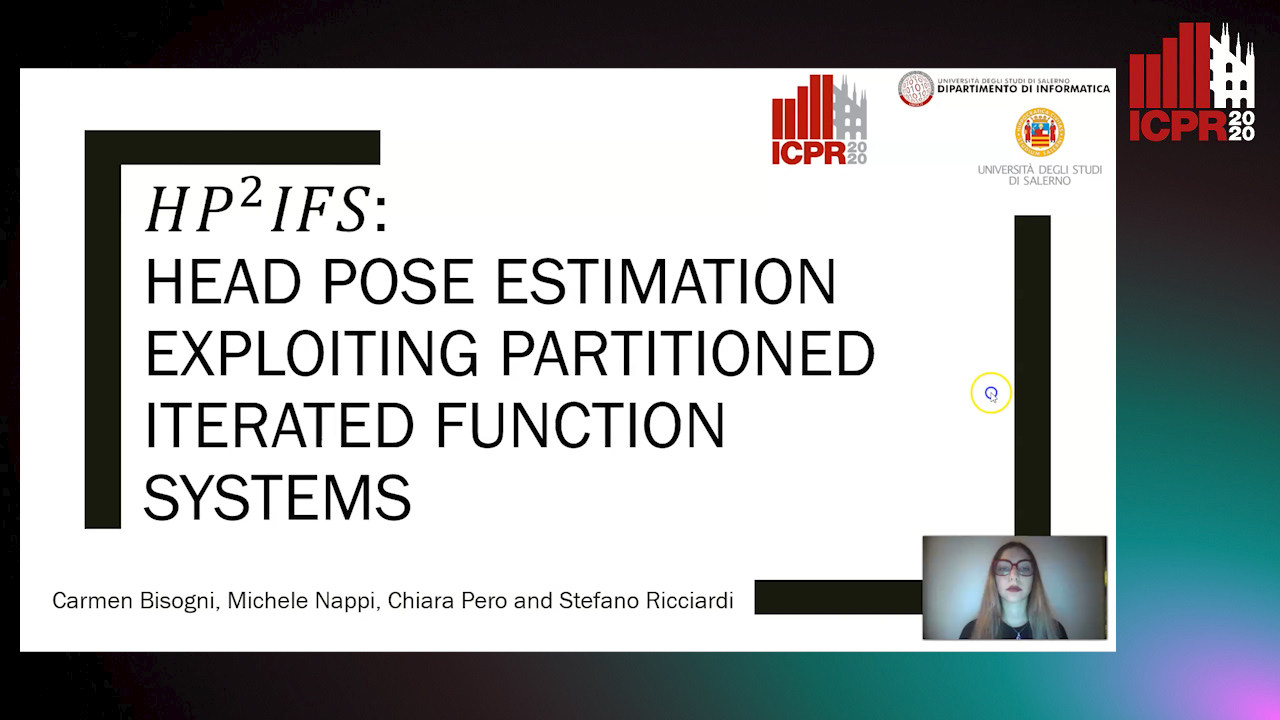
Auto-TLDR; PIFS based head pose estimation using fractal coding theory and Partitioned Iterated Function Systems
Abstract Slides Poster Similar
Estimating the actual head orientation from 2D images, with regard to its three degrees of freedom, is a well known problem that is highly significant for a large number of applications involving head pose knowledge. Consequently, this topic has been tackled by a plethora of methods and algorithms the most part of which exploits neural networks. Machine learning methods, indeed, achieve accurate head rotation values yet require an adequate training stage and, to that aim, a relevant number of positive and negative examples. In this paper we take a different approach to this topic by using fractal coding theory and particularly Partitioned Iterated Function Systems to extract the fractal code from the input head image and to compare this representation to the fractal code of a reference model through Hamming distance. According to experiments conducted on both the BIWI and the AFLW2000 databases, the proposed PIFS based head pose estimation method provides accurate yaw/pitch/roll angular values, with a performance approaching that of state of the art of machine-learning based algorithms and exceeding most of non-training based approaches.