Haibing Guan
Paper download is intended for registered attendees only, and is
subjected to the IEEE Copyright Policy. Any other use is strongly forbidden.
Papers from this author
VTT: Long-Term Visual Tracking with Transformers
Tianling Bian, Yang Hua, Tao Song, Zhengui Xue, Ruhui Ma, Neil Robertson, Haibing Guan
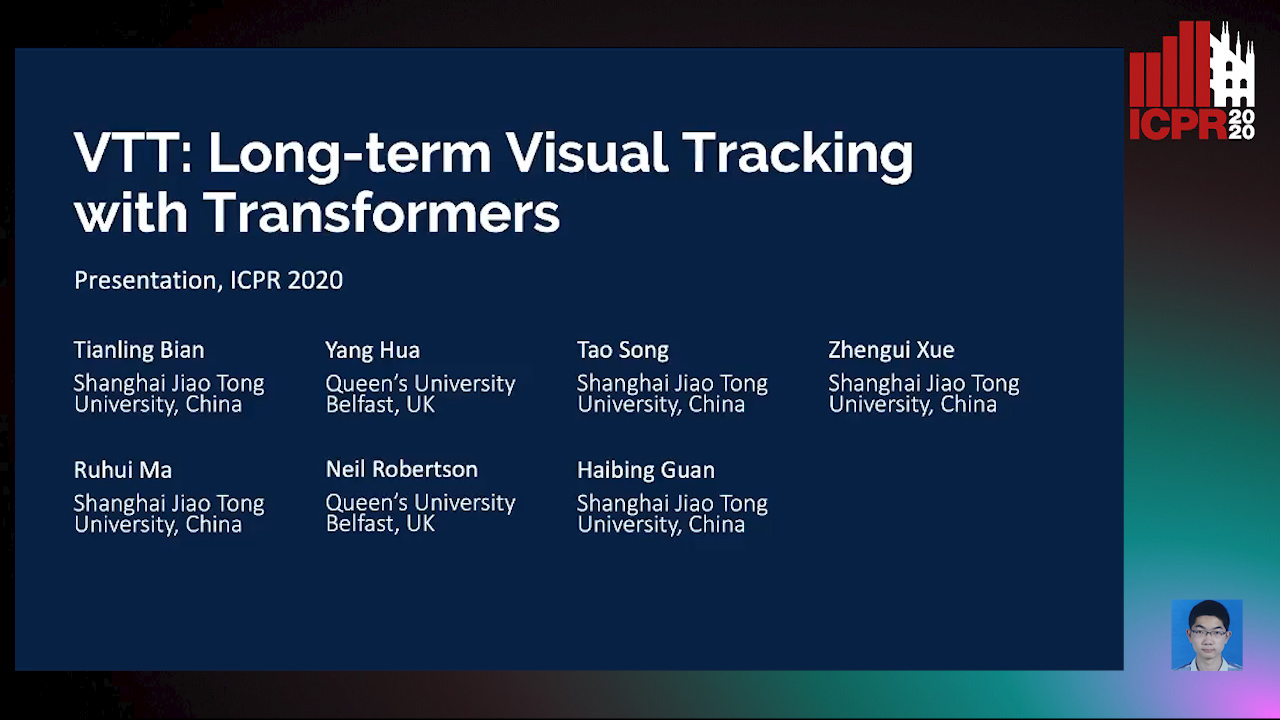
Auto-TLDR; Visual Tracking Transformer with transformers for long-term visual tracking
Long-term visual tracking is a challenging problem. State-of-the-art long-term trackers, e.g., GlobalTrack, utilize region proposal networks (RPNs) to generate target proposals. However, the performance of the trackers is affected by occlusions and large scale or ratio variations. To address these issues, in this paper, we are the first to propose a novel architecture with transformers for long-term visual tracking. Specifically, the proposed Visual Tracking Transformer (VTT) utilizes a transformer encoder-decoder architecture for aggregating global information to deal with occlusion and large scale or ratio variation. Furthermore, it also shows better discriminative power against instance-level distractors without the need for extra labeling and hard-sample mining. We conduct extensive experiments on three largest long-term tracking dataset and have achieved state-of-the-art performance.