Jang-Ho Choi
Paper download is intended for registered attendees only, and is
subjected to the IEEE Copyright Policy. Any other use is strongly forbidden.
Papers from this author
End-To-End Multi-Task Learning of Missing Value Imputation and Forecasting in Time-Series Data
Jinhee Kim, Taesung Kim, Jang-Ho Choi, Jaegul Choo
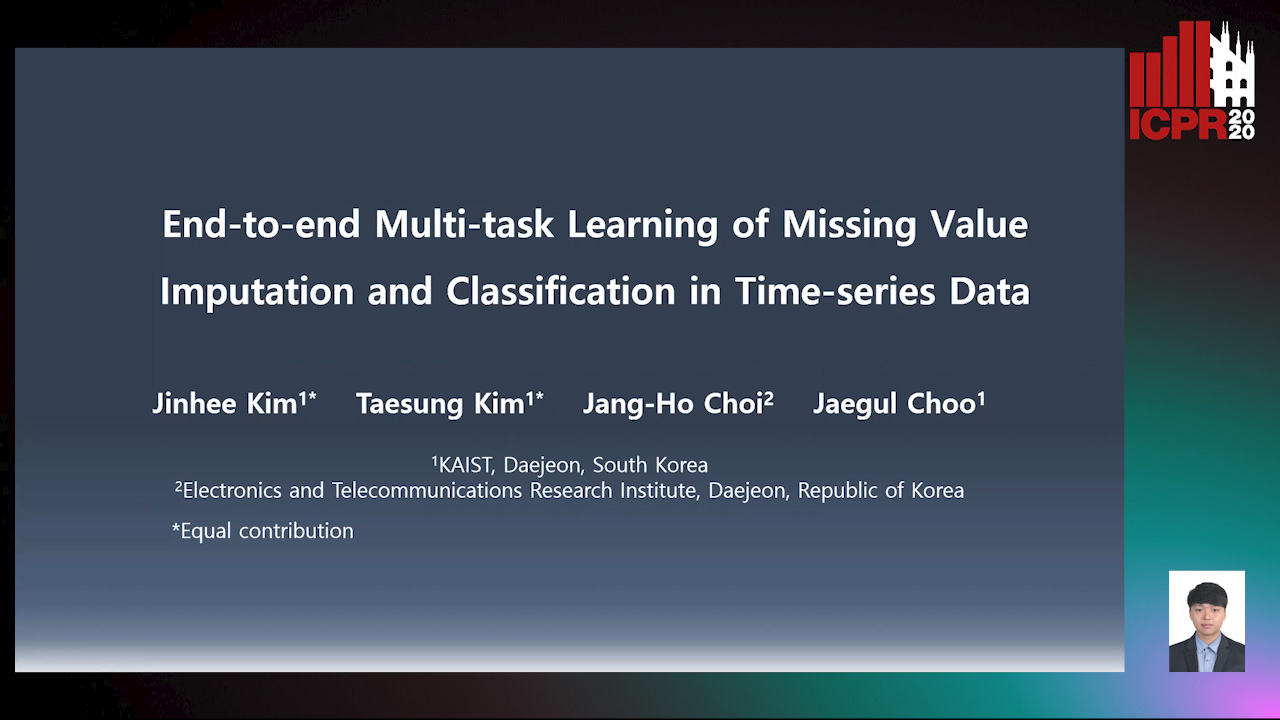
Auto-TLDR; Time-Series Prediction with Denoising and Imputation of Missing Data
Abstract Slides Poster Similar
Multivariate time-series prediction is a common task, but it often becomes challenging due to missing values involved in data caused by unreliable sensors and other issues. In fact, inaccurate imputation of missing values can degrade the downstream prediction performance, so it may be better not to rely on the estimated values of missing data. Furthermore, observed data may contain noise, so denoising them can be helpful for the main task at hand. In response, we propose a novel approach that can automatically utilize the optimal combination of the observed and the estimated values to generate not only complete, but also noise-reduced data by our own gating mechanism. We evaluate our model on real-world time-series datasets and achieved state-of-the-art performance, demonstrating that our method successfully handle the incomplete datasets. Moreover, we present in-depth studies using a carefully designed, synthetic multivariate time-series dataset to verify the effectiveness of the proposed model. The ablation studies and the experimental analysis of the proposed gating mechanism show that the proposed method works as an effective denoising as well as imputation method for time-series classification tasks.