Juntao Liang
Paper download is intended for registered attendees only, and is
subjected to the IEEE Copyright Policy. Any other use is strongly forbidden.
Papers from this author
Learning Semantic Representations Via Joint 3D Face Reconstruction and Facial Attribute Estimation
Zichun Weng, Youjun Xiang, Xianfeng Li, Juntao Liang, Wanliang Huo, Yuli Fu
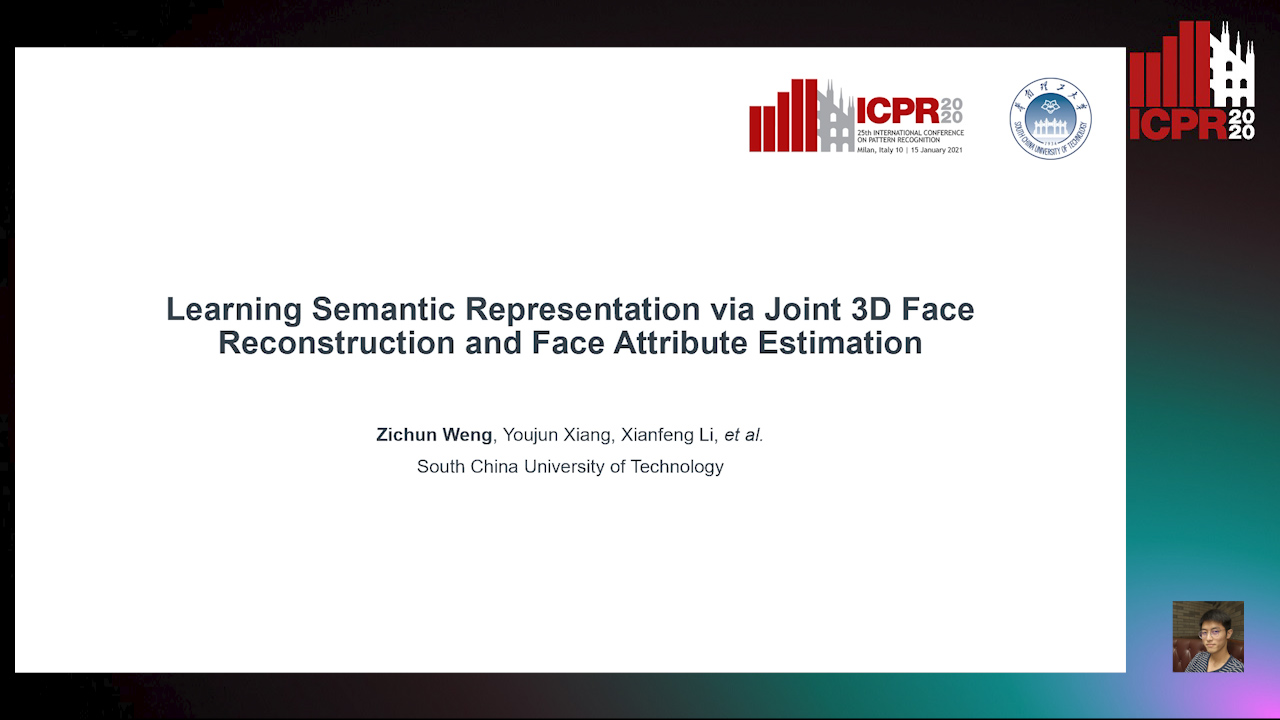
Auto-TLDR; Joint Framework for 3D Face Reconstruction with Facial Attribute Estimation
Abstract Slides Poster Similar
We propose a novel joint framework for 3D face reconstruction (3DFR) that integrates facial attribute estimation (FAE) as an auxiliary task. One of the essential problems of 3DFR is to extract semantic facial features (e.g., Big Nose, High Cheekbones, and Asian) from in-the-wild 2D images, which is inherently involved with FAE. These two tasks, though heterogeneous, are highly relevant to each other. To achieve this, we leverage a Convolutional Neural Network to extract shared facial representations for both shape decoder and attribute classifier. We further develop an in-batch hybrid-task training scheme that enables our model to learn from heterogeneous facial datasets jointly within a mini-batch. Thanks to the joint loss that provides supervision from both 3DFR and FAE domains, our model learns the correlations between 3D shapes and facial attributes, which benefit both feature extraction and shape inference. Quantitative evaluation and qualitative visualization results confirm the effectiveness and robustness of our joint framework.