Deep Yap Deep Yap
Paper download is intended for registered attendees only, and is
subjected to the IEEE Copyright Policy. Any other use is strongly forbidden.
Papers from this author
Answer-Checking in Context: A Multi-Modal Fully Attention Network for Visual Question Answering
Hantao Huang, Tao Han, Wei Han, Deep Yap Deep Yap, Cheng-Ming Chiang
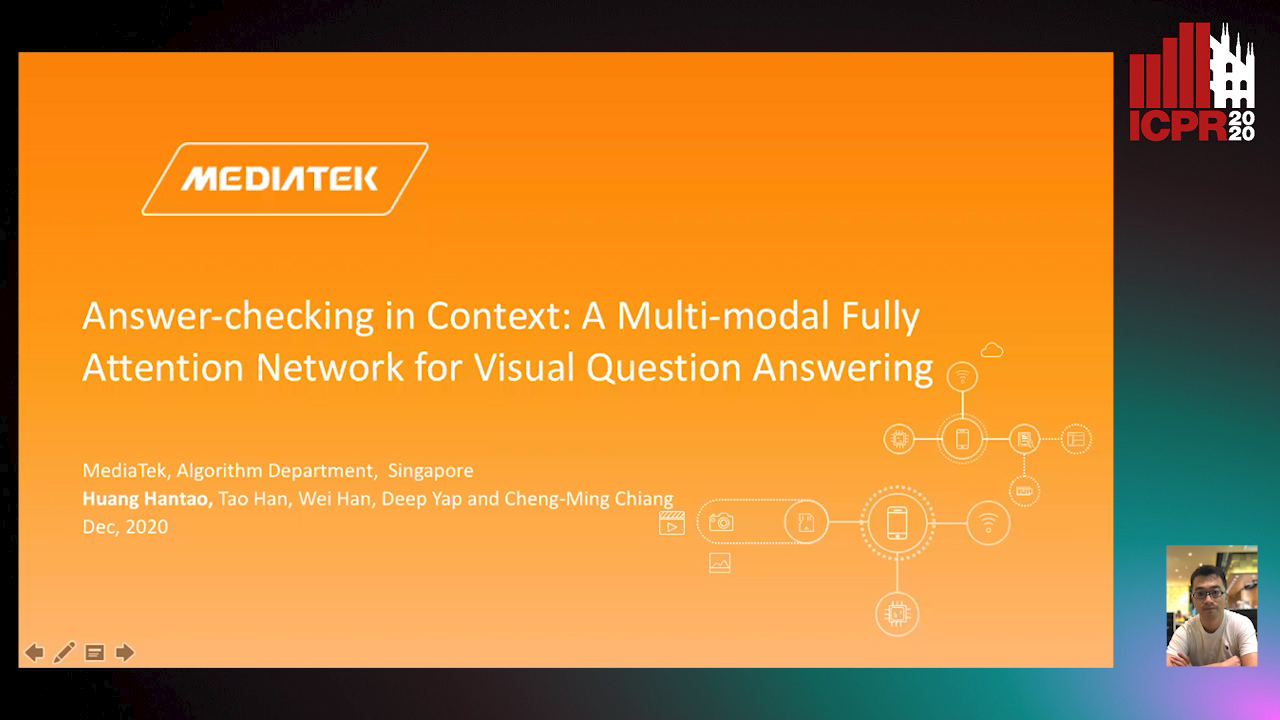
Auto-TLDR; Fully Attention Based Visual Question Answering
Abstract Slides Poster Similar
Visual Question Answering (VQA) is challenging due to the complex cross-modality relations. It has received extensive attention from the research community. From the human perspective, to answer a visual question, one needs to read the question and then refer to the image to generate an answer. Such answer will then be checked against the question and image again for the final confirmation. In this paper, we mimic this process and propose a fully attention based VQA architecture. Moreover, an answer-checking module is proposed to perform a unified attention on the jointly answer, question and image representation to update the answer. This mimics the human answer checking process to consider the answer in the context. With answer-checking modules and transferred BERT layers, our model achieves a state-of-the-art accuracy 71.57\% using less parameters on VQA-v2.0 test-standard split.