Xin Du
Paper download is intended for registered attendees only, and is
subjected to the IEEE Copyright Policy. Any other use is strongly forbidden.
Papers from this author
Automatical Enhancement and Denoising of Extremely Low-Light Images
Yuda Song, Yunfang Zhu, Xin Du
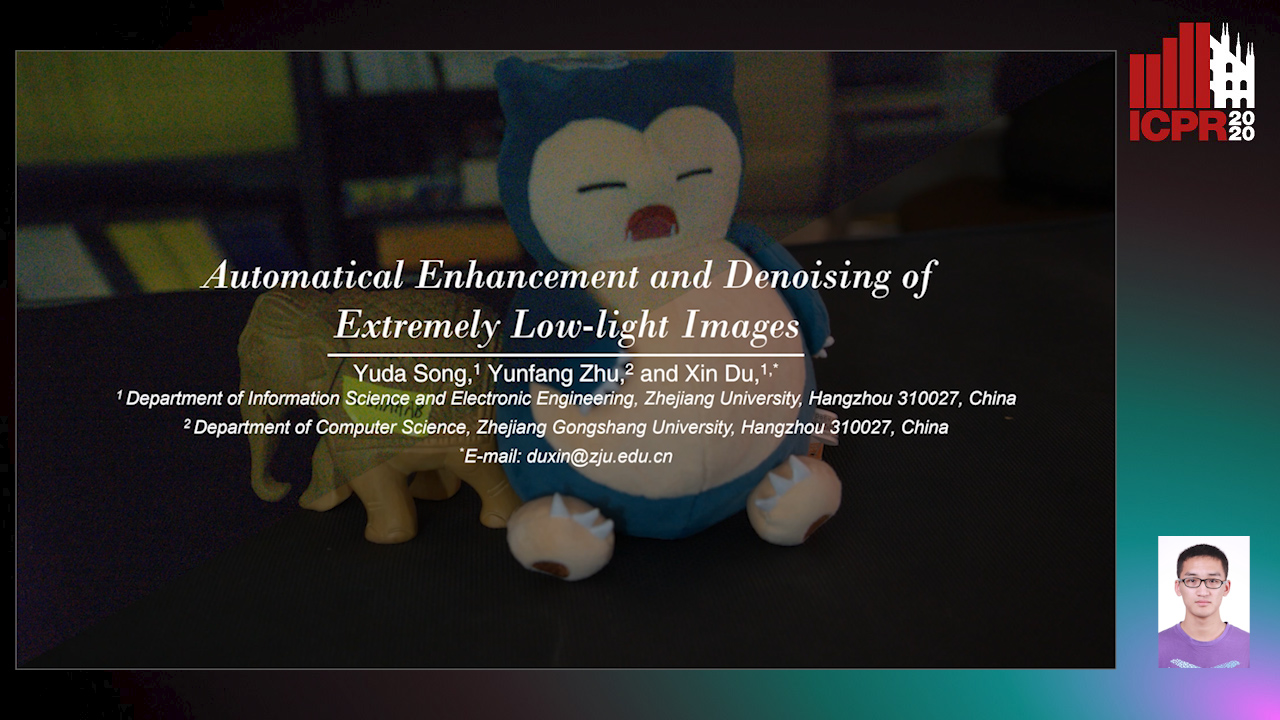
Auto-TLDR; INSNet: Illumination and Noise Separation Network for Low-Light Image Restoring
Abstract Slides Poster Similar
Deep convolutional neural networks (DCNN) based methodologies have achieved remarkable performance on various low-level vision tasks recently. Restoring images captured at night is one of the trickiest low-level vision tasks due to its high-level noise and low-level intensity. We propose a DCNN-based methodology, Illumination and Noise Separation Network (INSNet), which performs both denoising and enhancement on these extremely low-light images. INSNet fully utilizes global-ware features and local-ware features using the modified network structure and image sampling scheme. Compared to well-designed complex neural networks, our proposed methodology only needs to add a bypass network to the existing network. However, it can boost the quality of recovered images dramatically but only increase the computational cost by less than 0.1%. Even without any manual settings, INSNet can stably restore the extremely low-light images to desired high-quality images.