Dong Liang
Paper download is intended for registered attendees only, and is
subjected to the IEEE Copyright Policy. Any other use is strongly forbidden.
Papers from this author
Coarse-To-Fine Foreground Segmentation Based on Co-Occurrence Pixel-Block and Spatio-Temporal Attention Model
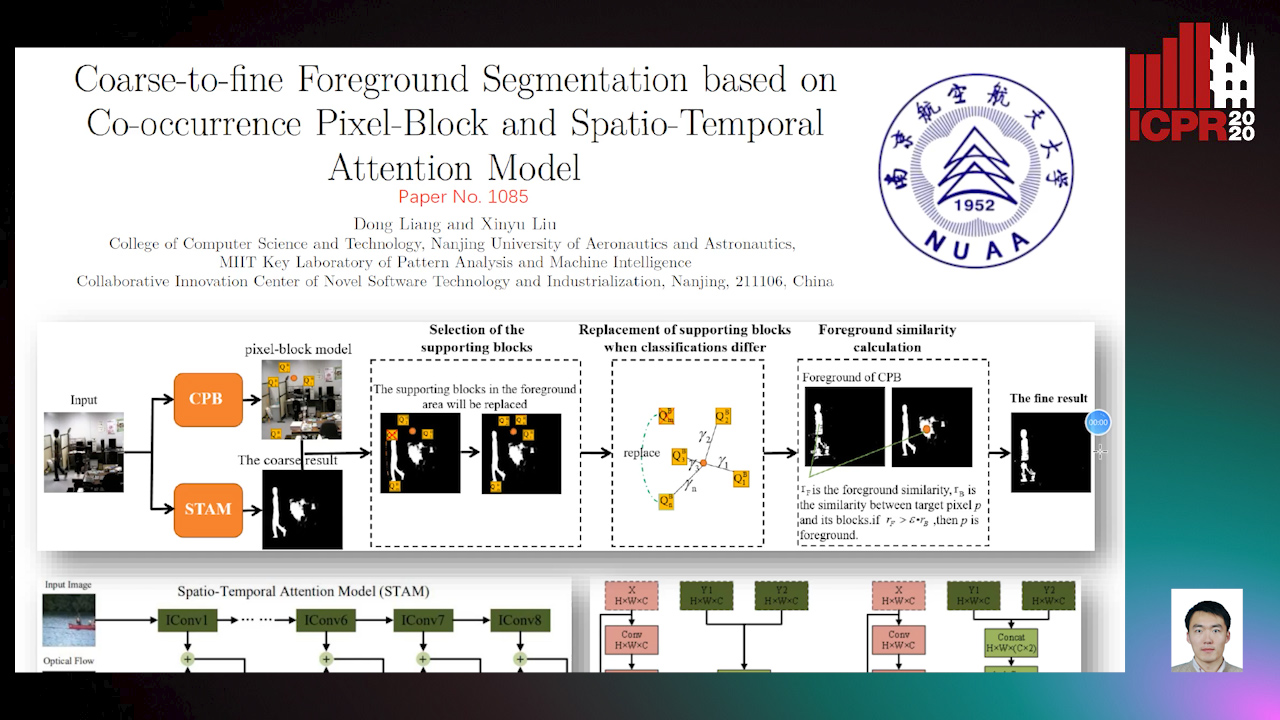
Auto-TLDR; Foreground Segmentation from coarse to Fine Using Co-occurrence Pixel-Block Model for Dynamic Scene
Abstract Slides Poster Similar
Foreground segmentation in dynamic scene is an important task in video surveillance. The unsupervised background subtraction method based on background statistics modeling has difficulties in updating. On the other hand, the supervised foreground segmentation method based on deep learning relies on the large-scale of accurately annotated training data, which limits its cross-scene performance. In this paper, we propose a foreground segmentation method from coarse to fine. First, a across-scenes trained Spatio-Temporal Attention Model (STAM) is used to achieve coarse segmentation, which does not require training on specific scene. Then the coarse segmentation is used as a reference to help Co-occurrence Pixel-Block Model (CPB) complete the fine segmentation, and at the same time help CPB to update its background model. This method is more flexible than those deep-learning-based methods which depends on the specific-scene training, and realizes the accurate online dynamic update of the background model. Experimental results on WallFlower and LIMU validate our method outperforms STAM, CPB and other methods of participating in comparison.